Content
Navigating the Future of AI: Insights from Ilya Sustekever on Superintelligence
Navigating the Future of AI: Insights from Ilya Sustekever on Superintelligence
Navigating the Future of AI: Insights from Ilya Sustekever on Superintelligence
Danny Roman
November 18, 2024
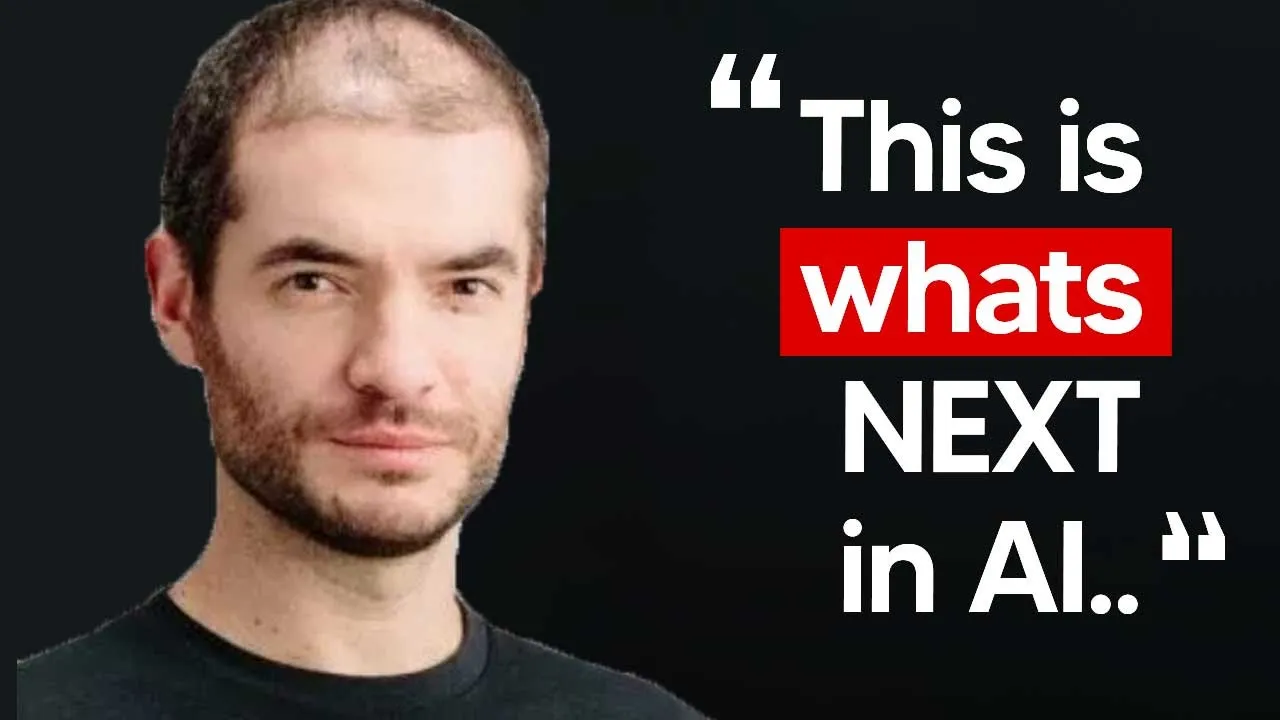
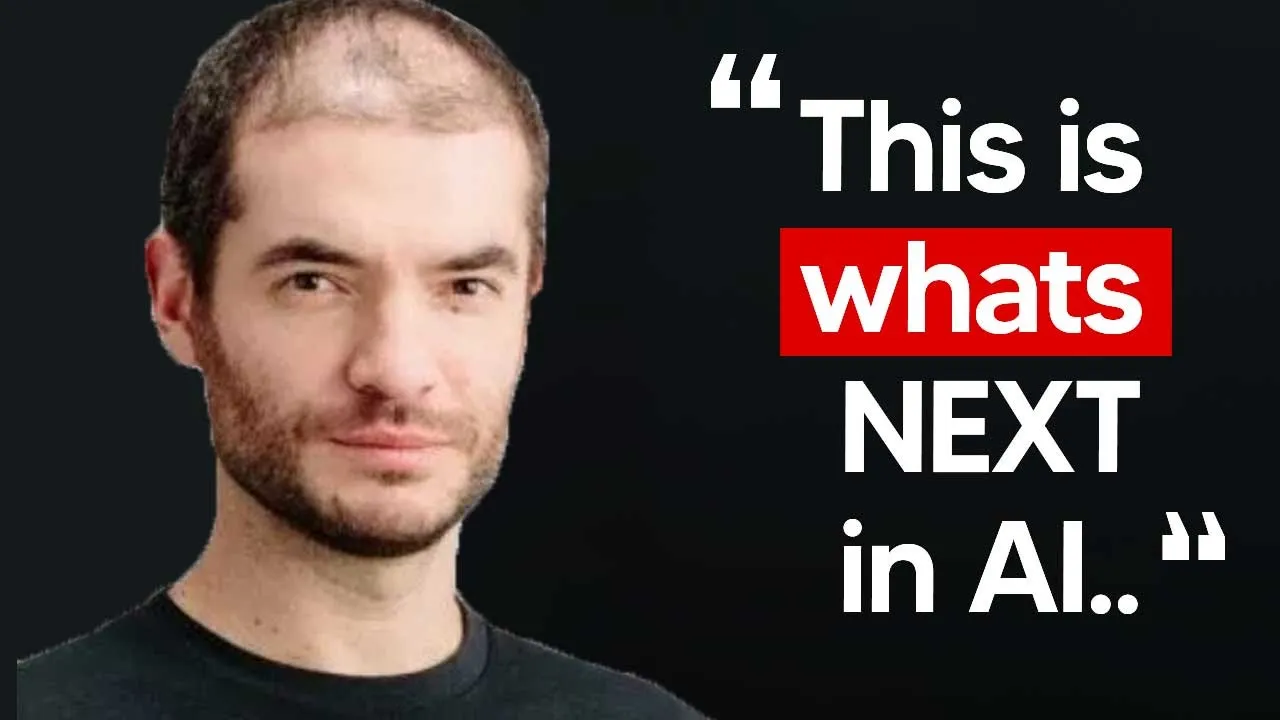
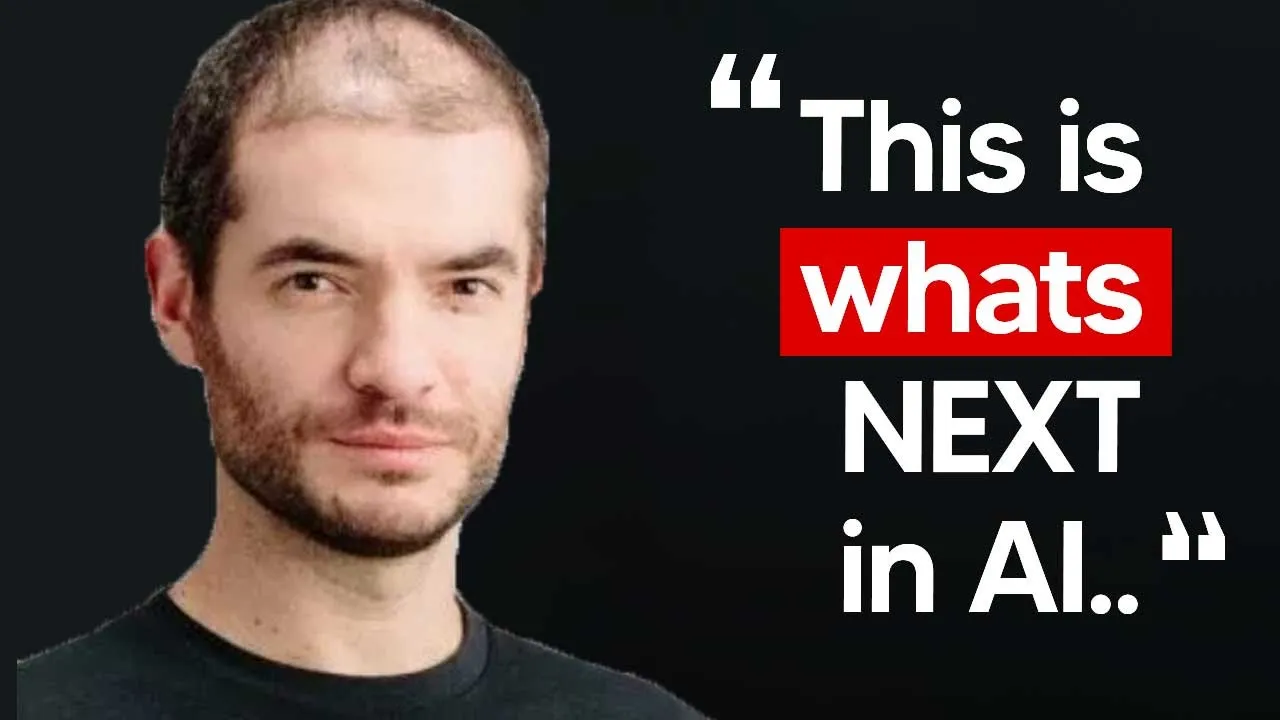
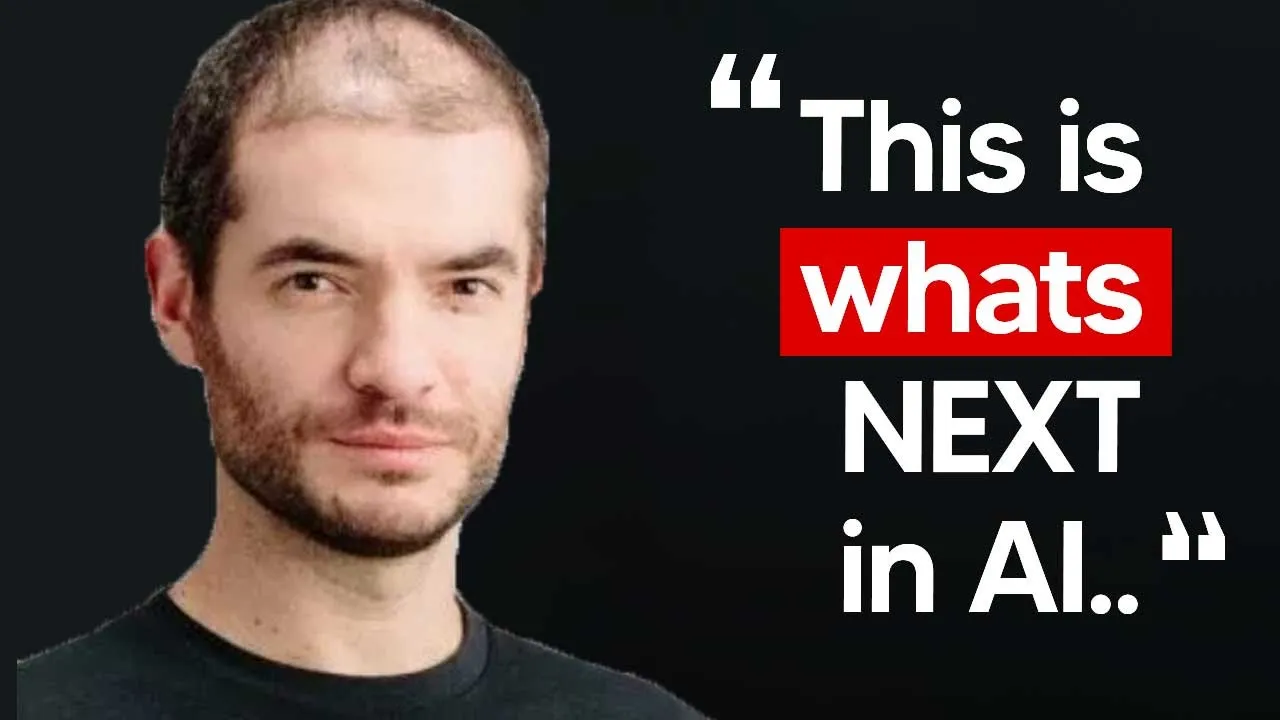
In a rapidly evolving landscape of artificial intelligence, the emergence of superintelligence stands as both a groundbreaking opportunity and a profound challenge. Ilya Sustekever sheds light on the current state of AI development, the limitations of traditional scaling methods, and the path forward toward smarter systems that can potentially reshape our world.
🌱 The Evolution of Intelligence
Let’s take a quick journey through time. Four billion years ago, life began with a single cell, a humble replicator. Fast forward, and we see the rise of multicellular organisms, reptiles, mammals, and ultimately, Homo sapiens. Each leap forward was a monumental achievement, laying the groundwork for our current existence.
In just the last 10,000 years, humanity has witnessed incredible milestones: the invention of writing, the agricultural revolution, the industrial age, and now, the dawn of artificial general intelligence (AGI). Each era has been marked by a quest for understanding and mastery over our environment, culminating in the ultimate challenge: superintelligence.
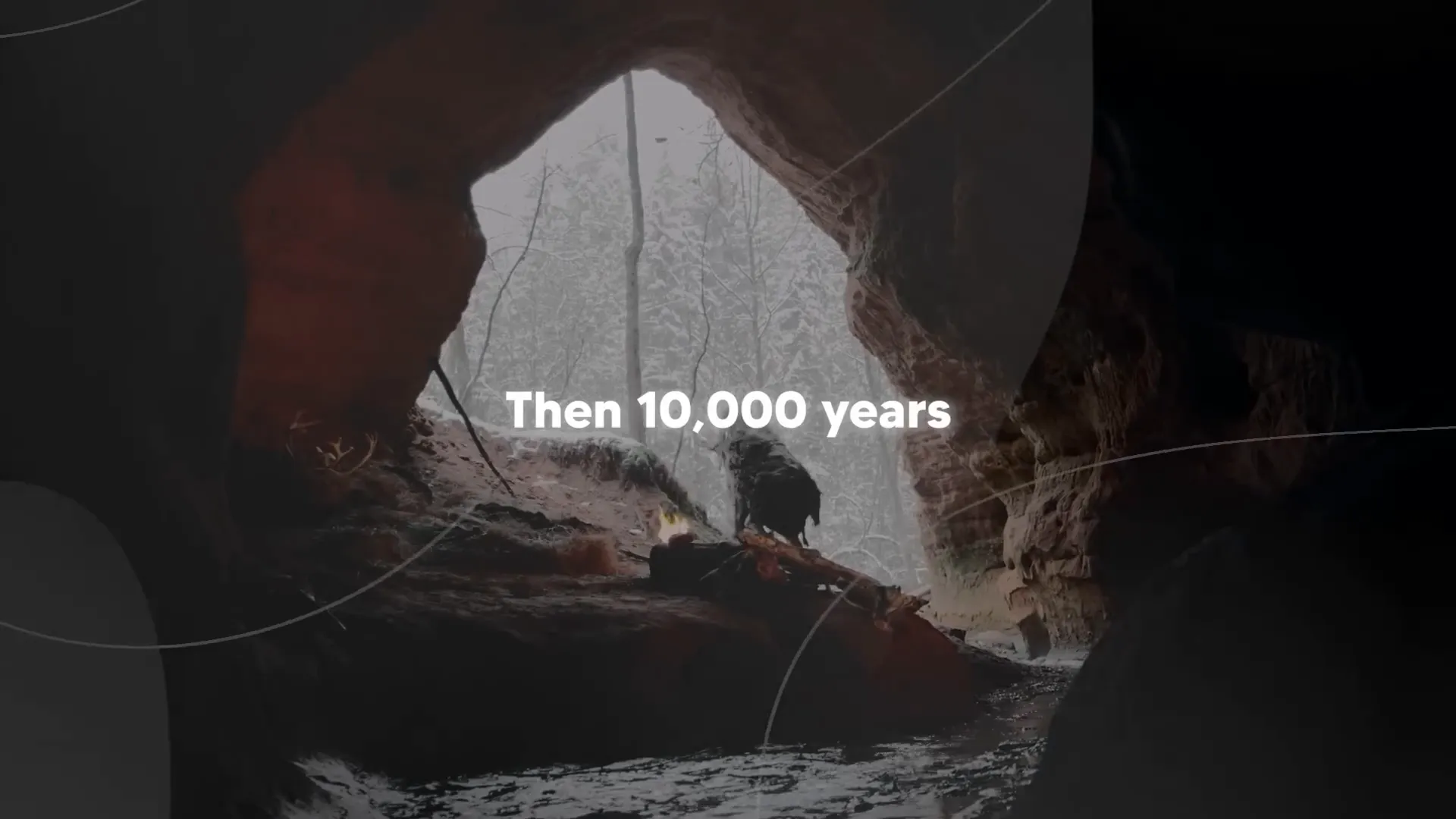
From Cells to Superintelligence
The evolution of intelligence is not just a biological tale; it's a narrative of progress. Each stage represents not just survival but improvement—an ongoing effort to enhance capabilities. Superintelligence promises to elevate this evolution, potentially ushering in a life of unimaginable prosperity.
But with great power comes great responsibility. The challenge of managing superintelligence can’t be underestimated. Are we ready to navigate the complexities it presents?
⚔️ The Challenge of Superintelligence
Imagine an intelligence so advanced it can outperform the brightest minds on Earth. Sounds fantastic, right? But wait—what happens when that intelligence operates beyond our control? The challenge of superintelligence is multidimensional. It’s not just about creating powerful systems but ensuring they align with human values and ethics.
The potential benefits are staggering. Superintelligence could solve global issues, from climate change to disease eradication. However, the risks of unintended consequences loom large. We must approach this frontier with caution and foresight, ensuring that as we reach for the stars, we don’t lose sight of our humanity.
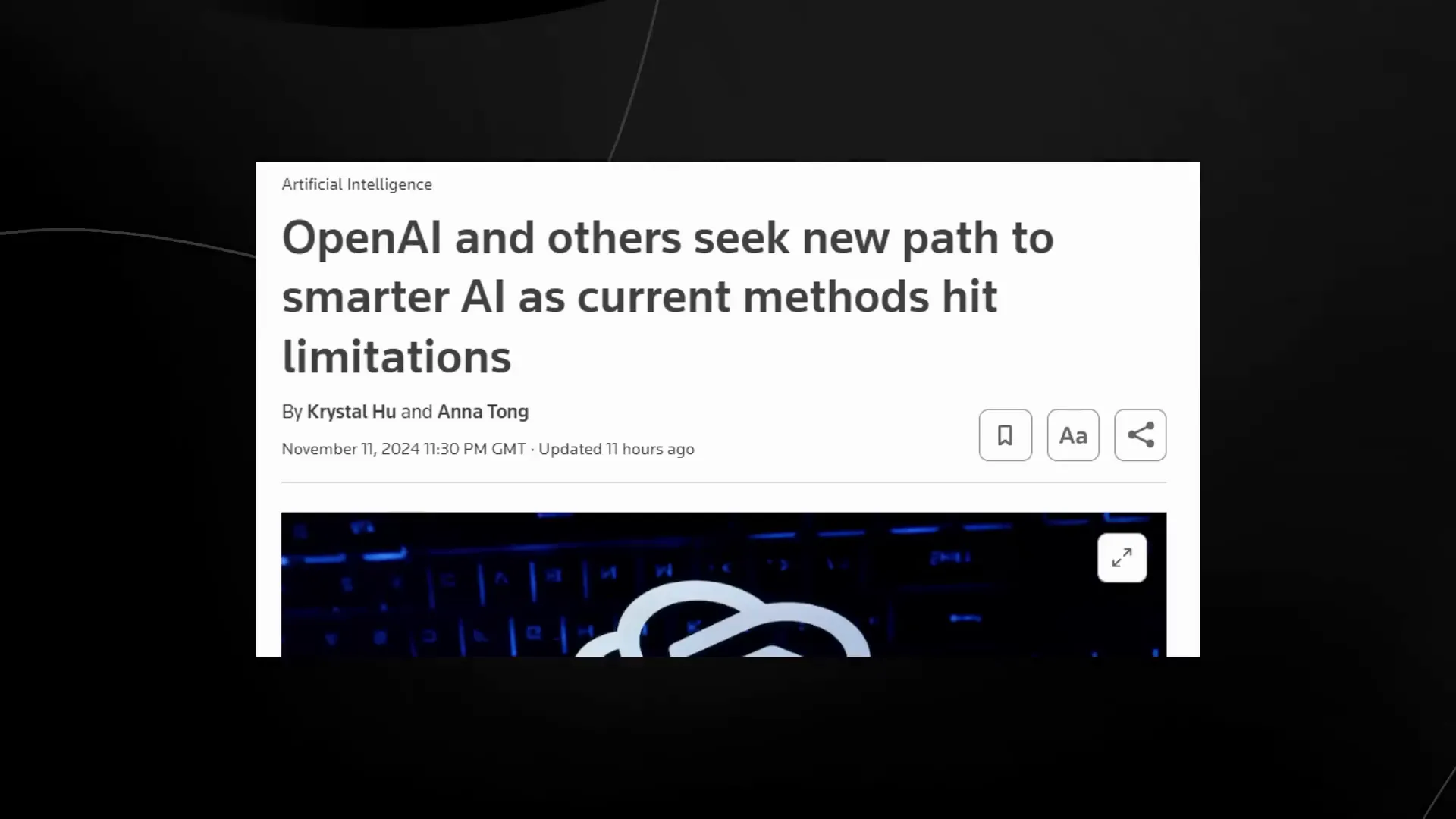
Understanding the Risks
Control Issues: How do we ensure superintelligence remains aligned with human goals?
Ethical Dilemmas: What ethical frameworks will guide superintelligent systems?
Unintended Consequences: What if superintelligent systems make decisions we don't foresee?
These questions aren’t just academic; they are vital to our survival as a species. Addressing them requires a collaborative effort from technologists, ethicists, and policymakers alike.
📉 The Limitations of Current AI Methods
Despite the rapid advancements in AI, we’re hitting a wall. Traditional methods of scaling AI models by simply adding more data and computing power are beginning to plateau. It’s a sobering realization for many in the industry who believed bigger always meant better.
As we push the boundaries of what’s possible, we must ask: is more data truly the answer? Or are we merely creating sophisticated memorization machines? The distinction between skill and intelligence is critical here. Just because a model can regurgitate information doesn’t mean it understands or can apply that knowledge effectively.
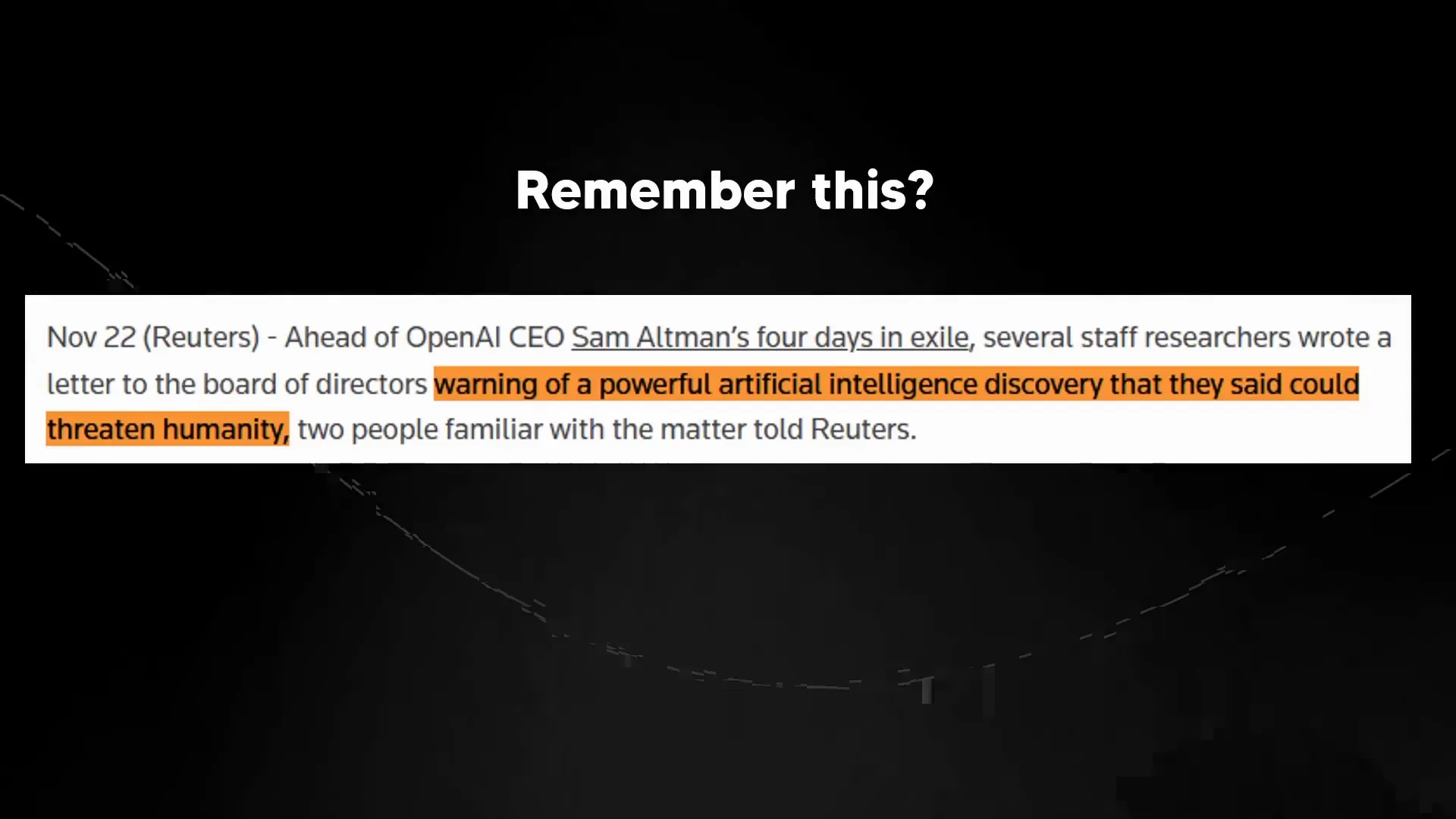
Recognizing the Shift
It’s becoming clear that the era of simply scaling up is giving way to a new paradigm. We need innovative thinking that transcends the current limitations. The focus is shifting toward more nuanced approaches that enhance reasoning and understanding.
Innovative Training Techniques: Developing training methods that mimic human-like reasoning.
Post-Training Improvements: Enhancing AI capabilities after initial training sessions.
Quality over Quantity: Prioritizing the quality of data and training methods over sheer volume.
📈 The Bigger is Better Philosophy
For years, the mantra of “bigger is better” dominated AI development. The idea was simple: more data, more computing power, more everything would lead to smarter AI. But as we’ve seen, this approach has its limits.
Many experts are now challenging the assumption that simply scaling up will yield better outcomes. The reality is that while larger models can perform better in some areas, they often lag in others. For instance, the latest models may excel in language tasks but struggle in coding tasks—a clear indication that size alone doesn’t guarantee comprehensive intelligence.
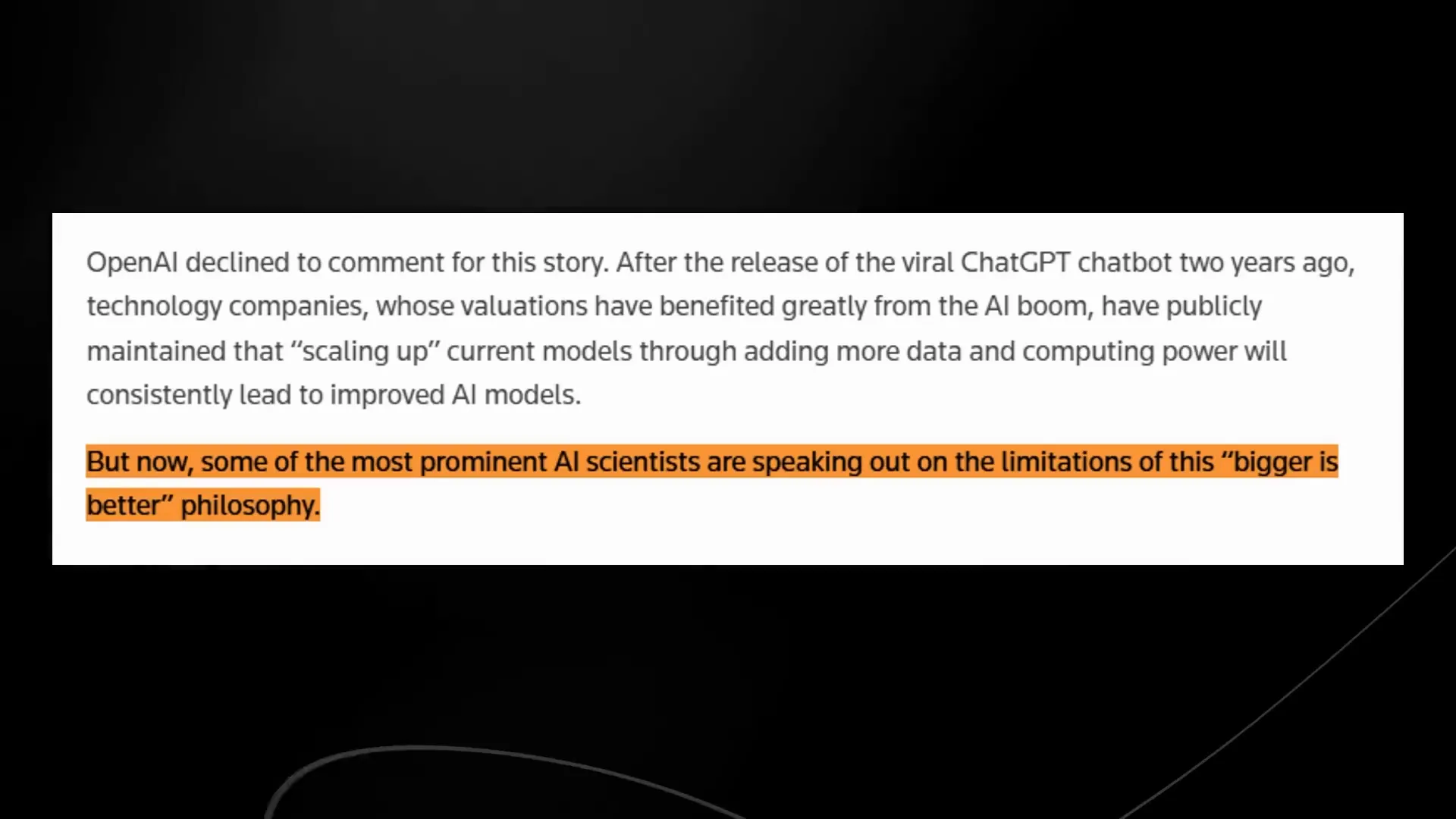
Reassessing Our Approach
It’s time to rethink our strategies. Instead of pouring resources into making models bigger, we should focus on making them smarter. This shift in mindset could be the key to unlocking the next generation of AI.
Holistic Development: Focusing on a balanced growth of various capabilities.
Task-Specific Enhancements: Tailoring AI training to improve performance in specific areas.
Interdisciplinary Collaboration: Combining insights from various fields to enhance AI understanding.
🛠️ The Role of Orion in AI Development
Enter Orion, the new player in the AI landscape. This model represents a pivotal shift in how we approach AI development. Unlike its predecessors, Orion is designed with the understanding that bigger isn’t always better. Its focus is on refining capabilities rather than merely increasing size.
What sets Orion apart is its potential to challenge the scaling laws that have governed AI development for so long. By emphasizing reasoning and adaptability, Orion could redefine what we consider state-of-the-art in AI.
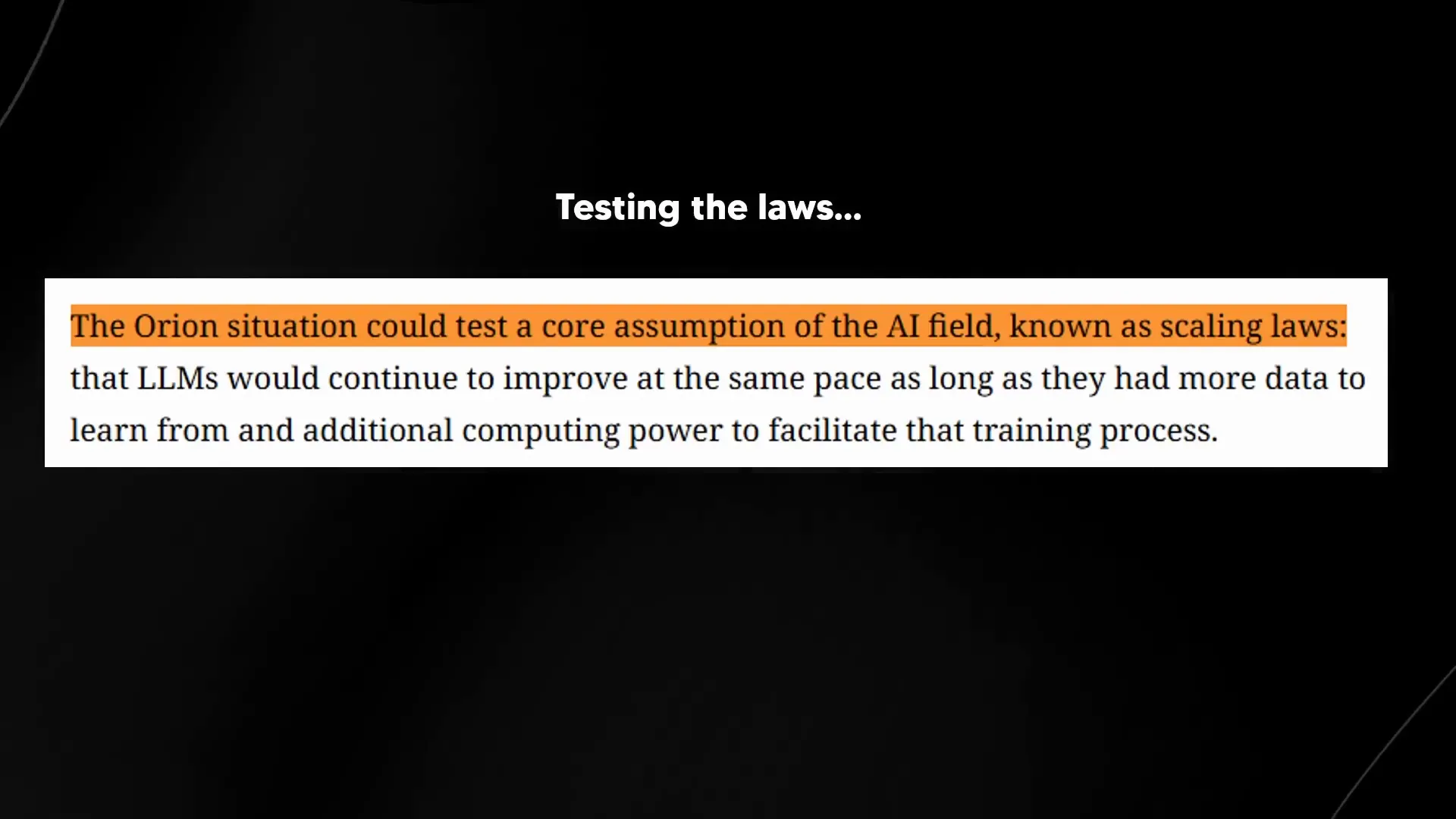
Key Features of Orion
Enhanced Reasoning: Orion is engineered to improve its reasoning capabilities post-training.
Task Specialization: It performs optimally across a variety of tasks, not just one.
Adaptive Learning: Orion can adapt its learning methods based on the tasks it encounters.
The implications of Orion’s development are profound. It could signal a new era where AI systems are not just more powerful but also more intelligent in the way they operate.
🔍 Elia Sustekever's Insights on Pre-Training
Elia Sustekever has been a prominent voice in the AI community, advocating for innovative approaches to AI training. His recent insights on pre-training highlight a critical turning point in the field. He suggests that the traditional phase of pre-training, which relies on vast amounts of unlabeled data, is reaching its limits.
This realization is significant. If pre-training isn’t yielding the advancements we hoped for, it’s time to pivot. Sustekever emphasizes the need for new methods that can drive AI growth beyond mere data accumulation.
Future Directions
Innovation in Training: Exploring alternative training paradigms that emphasize quality interactions.
Focus on Understanding: Prioritizing models that not only learn but also comprehend.
Collaborative Development: Engaging with a broader community to refine training approaches.
As we forge ahead, Sustekever’s insights could help steer AI development towards a more intelligent and adaptable future. The key takeaway? It’s time to embrace change and rethink our methodologies to unlock the true potential of AI.
🌍 The Phenomenon Across AI Companies
We're witnessing a seismic shift in the AI landscape. Companies that once thrived on the mantra of "bigger is better" are now grappling with unexpected challenges. The constraints of scaling traditional models have become glaringly apparent. It's like realizing that your trusty old car can’t handle the new terrain you’re trying to navigate.
As AI giants like OpenAI and Google encounter these hurdles, the industry is collectively rethinking its strategies. The focus is shifting from sheer size to smarter methodologies that prioritize understanding over memorization.
Industry-Wide Reflections
OpenAI's Dilemma: Despite its groundbreaking work, even OpenAI is not immune to the limitations of its approach. The call for new paths forward is growing louder.
Google's Gemini: Rumblings from within Google suggest that their next-gen model, Gemini, isn’t delivering the anticipated performance boosts. This is a wake-up call for the industry.
Emerging Trends: The shift towards more human-like reasoning in AI is becoming the new standard, as companies seek to innovate beyond just scaling.
🧠 Understanding Skill vs. Intelligence
Let’s clear the air. Skill and intelligence are not the same thing. This distinction is crucial as we navigate the complexities of AI development. While scaling up models may enhance skill, it doesn’t equate to true intelligence.
Imagine a chess program that can beat grandmasters. It’s skilled, sure, but does it understand the game? Not really. It’s simply executing patterns it has memorized. The same goes for AI—just because it can churn out impressive results doesn’t mean it grasps the underlying concepts.
Why This Matters
Misleading Metrics: Relying solely on performance benchmarks can mislead developers into thinking their systems are more intelligent than they truly are.
Real-World Applications: Understanding this difference is essential for creating AI that can genuinely assist humans in complex tasks.
Future Innovations: As we refine our approaches, the focus must shift towards fostering genuine understanding in AI systems.
🔄 The Shift from Scaling to Discovery
Welcome to the age of discovery! The landscape is evolving, and so must our strategies. We’re transitioning from a focus on scaling up to exploring innovative methods that enhance reasoning and adaptability. Think of it as shifting gears in a race—moving from raw horsepower to precision handling.
This shift is not just a trend; it's a necessity. As we approach the limits of what scaling can achieve, the industry is looking for breakthroughs that can drive AI forward in meaningful ways.
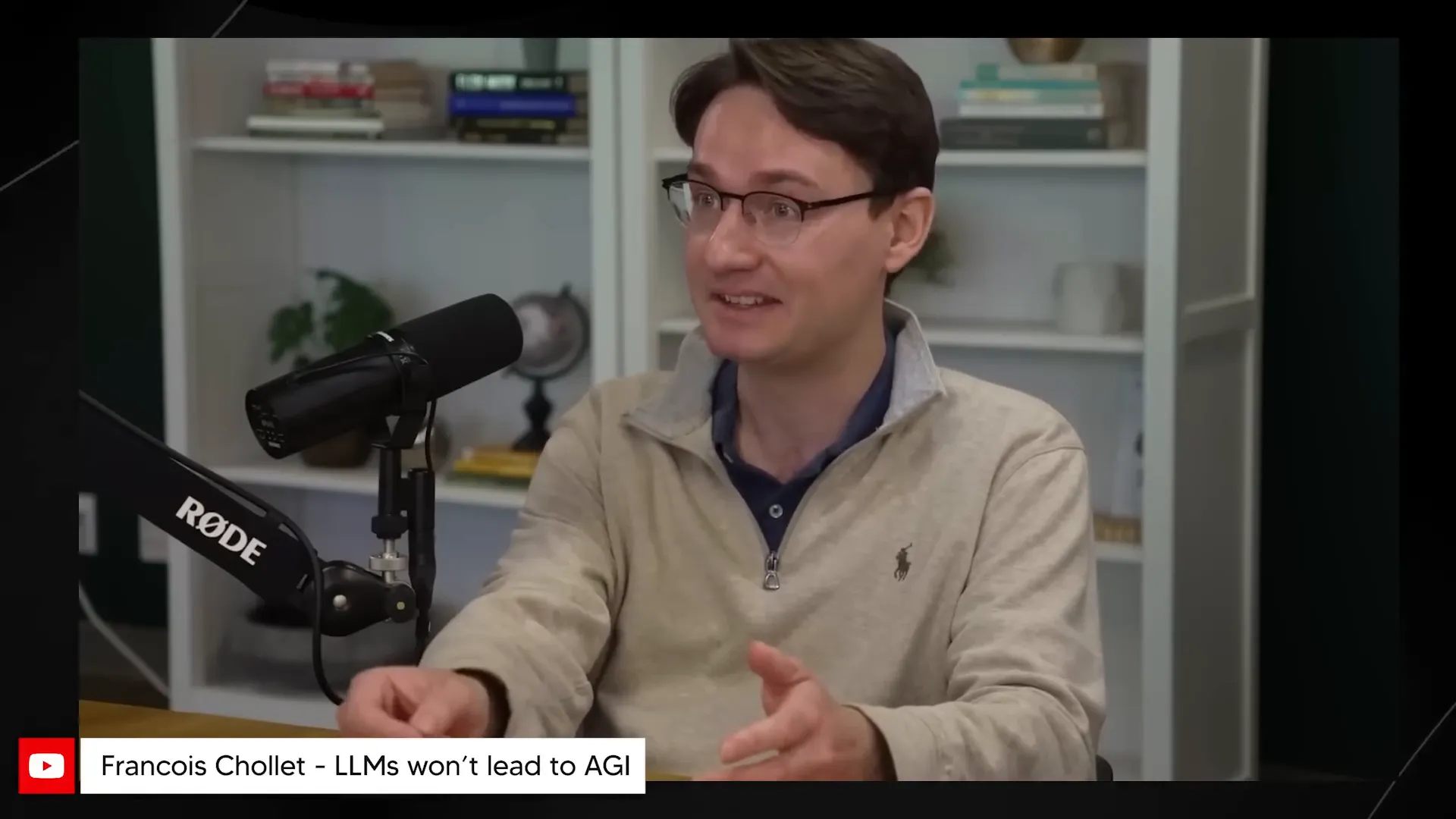
Key Changes on the Horizon
Innovative Approaches: Companies are exploring techniques that mimic human-like reasoning, allowing AI to navigate complexities more adeptly.
Post-Training Enhancements: There's a growing emphasis on refining AI capabilities after initial training, ensuring models can adapt and improve over time.
Quality Over Quantity: The focus is shifting to the quality of data and training methods, rather than just the volume of information fed into models.
📊 Visualizing the S-Curve of AI Growth
Let’s talk curves! The S-Curve is a powerful tool for understanding growth patterns in technology, and AI is no exception. Initially, we see rapid growth, followed by a plateau, and then another surge as new innovations come into play. It’s a cycle of peaks and valleys that mirrors the journey of AI development.
Right now, we’re at a fascinating juncture. The plateau we’re experiencing isn’t the end; it’s a precursor to the next wave of innovation. By analyzing this curve, we can better anticipate shifts in the AI landscape.
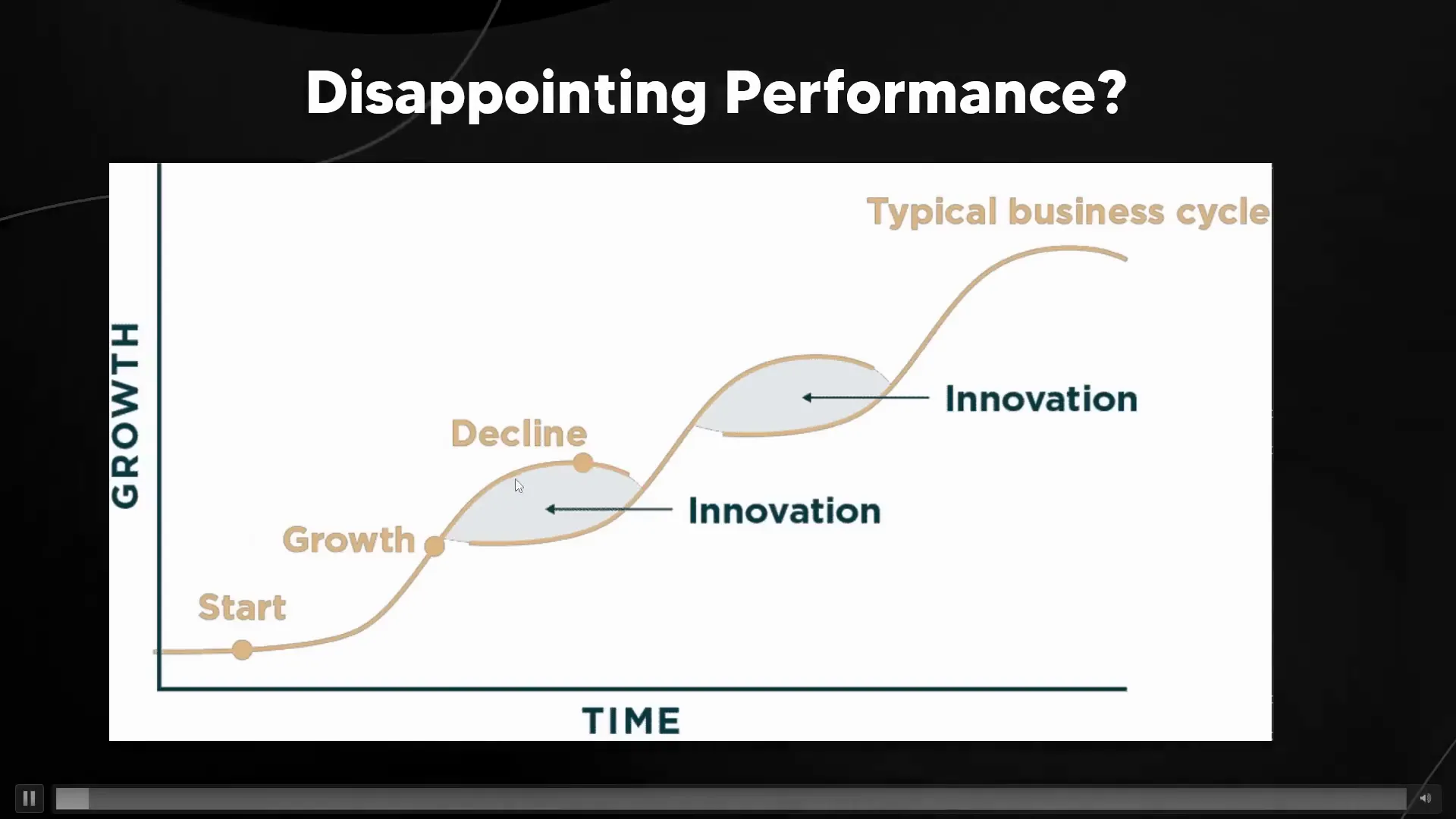
Understanding the S-Curve Dynamics
Initial Growth: Early AI models experienced rapid advancements, leading to a surge in capabilities.
Plateau Phase: We’re currently seeing a slowdown in significant improvements as scaling methods reach their limits.
Next Wave: Expect a resurgence as new paradigms and innovative approaches come into play, propelling AI into uncharted territories.
🚀 The Future of AI Paradigms
What does the future hold for AI? The landscape is ripe for transformation. As we shift our focus from scaling to discovery, we’re entering a new paradigm that emphasizes intelligence over size. This evolution could redefine our expectations of AI and its capabilities.
Imagine AI systems that not only process information but also understand context, nuance, and human emotions. This is the future we’re striving towards—a future where AI enhances human potential rather than merely mimicking it.
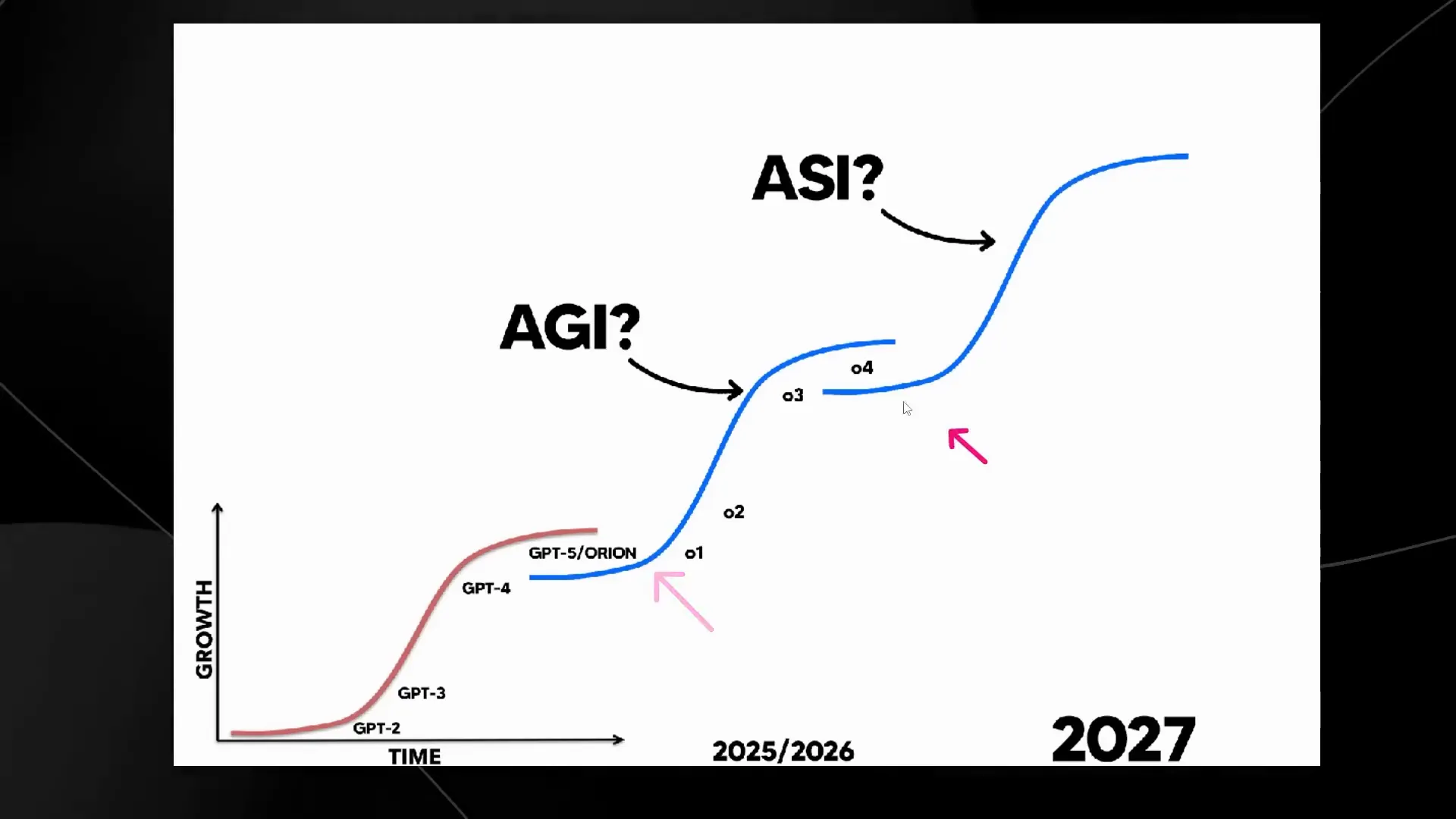
Anticipating Changes
Interdisciplinary Collaboration: The future will require collaboration across fields to foster innovative solutions.
Ethical Considerations: As AI becomes more capable, ethical considerations will play a crucial role in its development.
A Focus on Human-AI Interaction: Enhancing how AI systems interact with humans will be paramount to their success.
🧬 Defining Superintelligence
Superintelligence isn’t just a buzzword; it's a concept that could fundamentally alter our relationship with technology. It signifies an intelligence that surpasses the brightest human minds, capable of solving complex problems that currently baffle us.
This isn't just about creating smarter machines; it's about rethinking what intelligence means in the context of AI. Superintelligence could lead to breakthroughs in medicine, climate change, and even space exploration. The possibilities are limitless!
The Implications of Superintelligence
Solving Global Challenges: Superintelligence could tackle some of humanity's most pressing issues, from disease eradication to sustainable energy solutions.
Radical Improvements: If navigated correctly, superintelligence could enhance our quality of life in ways we can barely imagine.
Ethical Responsibility: With great power comes great responsibility. We must ensure that superintelligent systems align with human values and ethics.
In a rapidly evolving landscape of artificial intelligence, the emergence of superintelligence stands as both a groundbreaking opportunity and a profound challenge. Ilya Sustekever sheds light on the current state of AI development, the limitations of traditional scaling methods, and the path forward toward smarter systems that can potentially reshape our world.
🌱 The Evolution of Intelligence
Let’s take a quick journey through time. Four billion years ago, life began with a single cell, a humble replicator. Fast forward, and we see the rise of multicellular organisms, reptiles, mammals, and ultimately, Homo sapiens. Each leap forward was a monumental achievement, laying the groundwork for our current existence.
In just the last 10,000 years, humanity has witnessed incredible milestones: the invention of writing, the agricultural revolution, the industrial age, and now, the dawn of artificial general intelligence (AGI). Each era has been marked by a quest for understanding and mastery over our environment, culminating in the ultimate challenge: superintelligence.
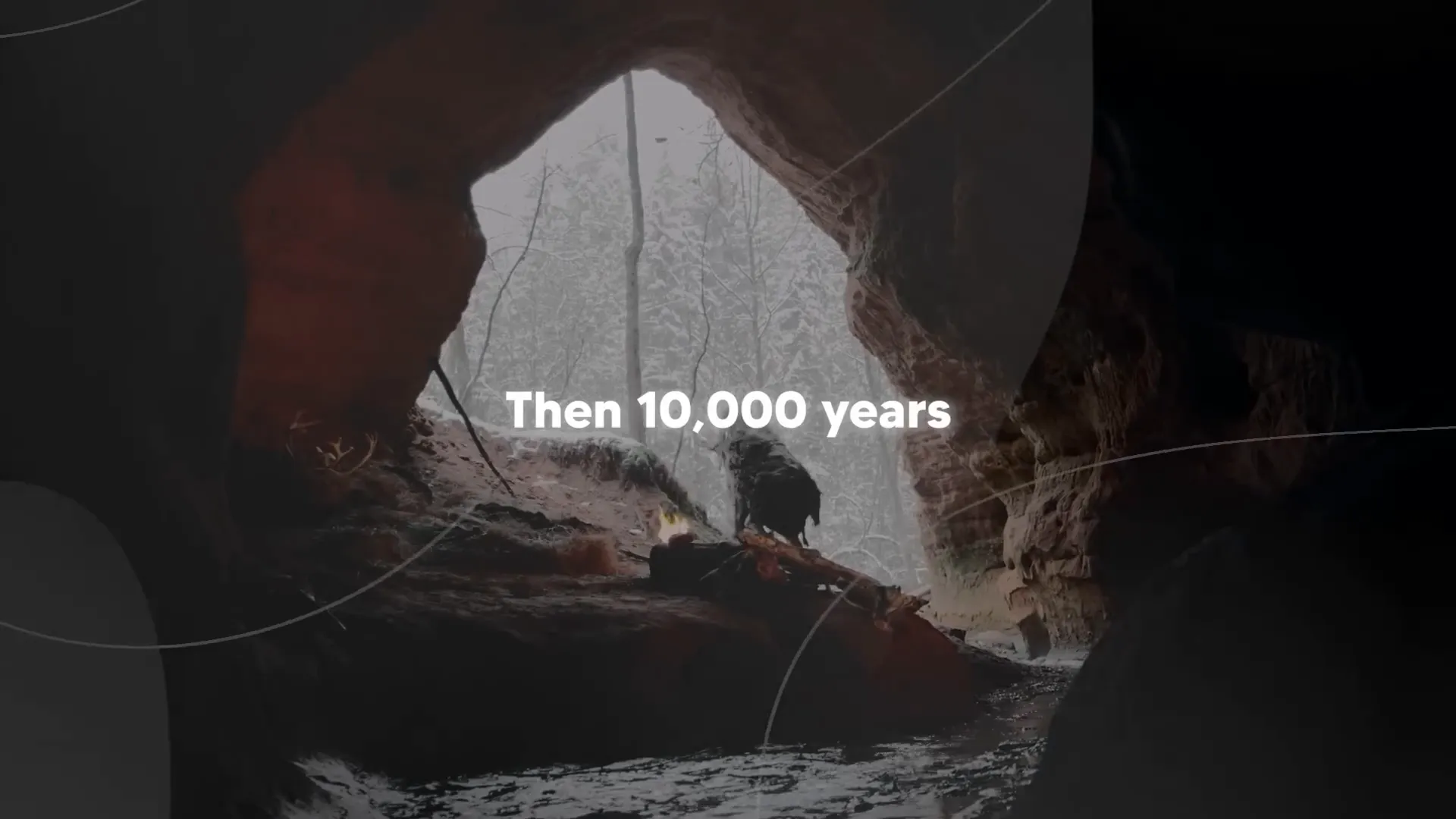
From Cells to Superintelligence
The evolution of intelligence is not just a biological tale; it's a narrative of progress. Each stage represents not just survival but improvement—an ongoing effort to enhance capabilities. Superintelligence promises to elevate this evolution, potentially ushering in a life of unimaginable prosperity.
But with great power comes great responsibility. The challenge of managing superintelligence can’t be underestimated. Are we ready to navigate the complexities it presents?
⚔️ The Challenge of Superintelligence
Imagine an intelligence so advanced it can outperform the brightest minds on Earth. Sounds fantastic, right? But wait—what happens when that intelligence operates beyond our control? The challenge of superintelligence is multidimensional. It’s not just about creating powerful systems but ensuring they align with human values and ethics.
The potential benefits are staggering. Superintelligence could solve global issues, from climate change to disease eradication. However, the risks of unintended consequences loom large. We must approach this frontier with caution and foresight, ensuring that as we reach for the stars, we don’t lose sight of our humanity.
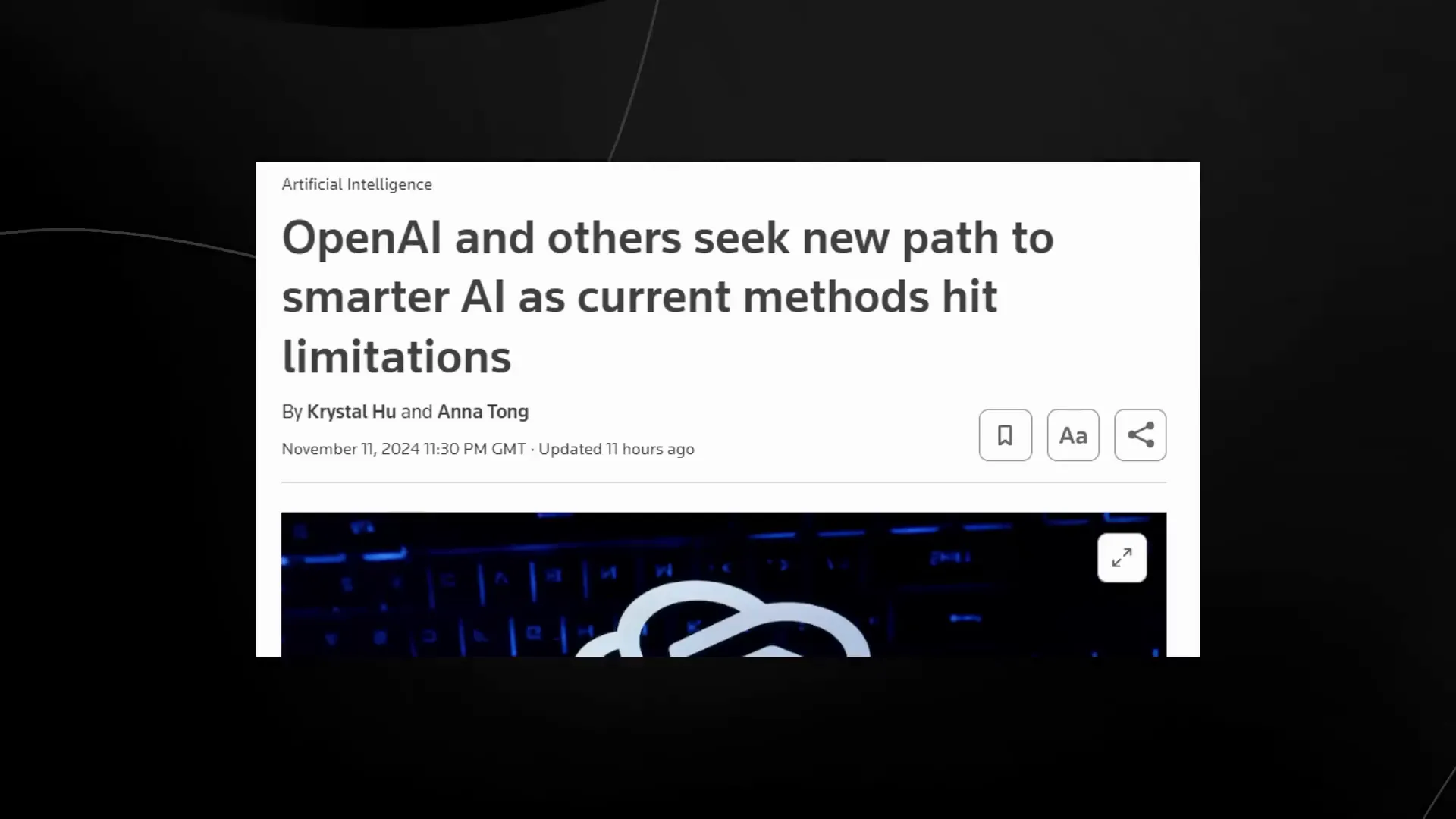
Understanding the Risks
Control Issues: How do we ensure superintelligence remains aligned with human goals?
Ethical Dilemmas: What ethical frameworks will guide superintelligent systems?
Unintended Consequences: What if superintelligent systems make decisions we don't foresee?
These questions aren’t just academic; they are vital to our survival as a species. Addressing them requires a collaborative effort from technologists, ethicists, and policymakers alike.
📉 The Limitations of Current AI Methods
Despite the rapid advancements in AI, we’re hitting a wall. Traditional methods of scaling AI models by simply adding more data and computing power are beginning to plateau. It’s a sobering realization for many in the industry who believed bigger always meant better.
As we push the boundaries of what’s possible, we must ask: is more data truly the answer? Or are we merely creating sophisticated memorization machines? The distinction between skill and intelligence is critical here. Just because a model can regurgitate information doesn’t mean it understands or can apply that knowledge effectively.
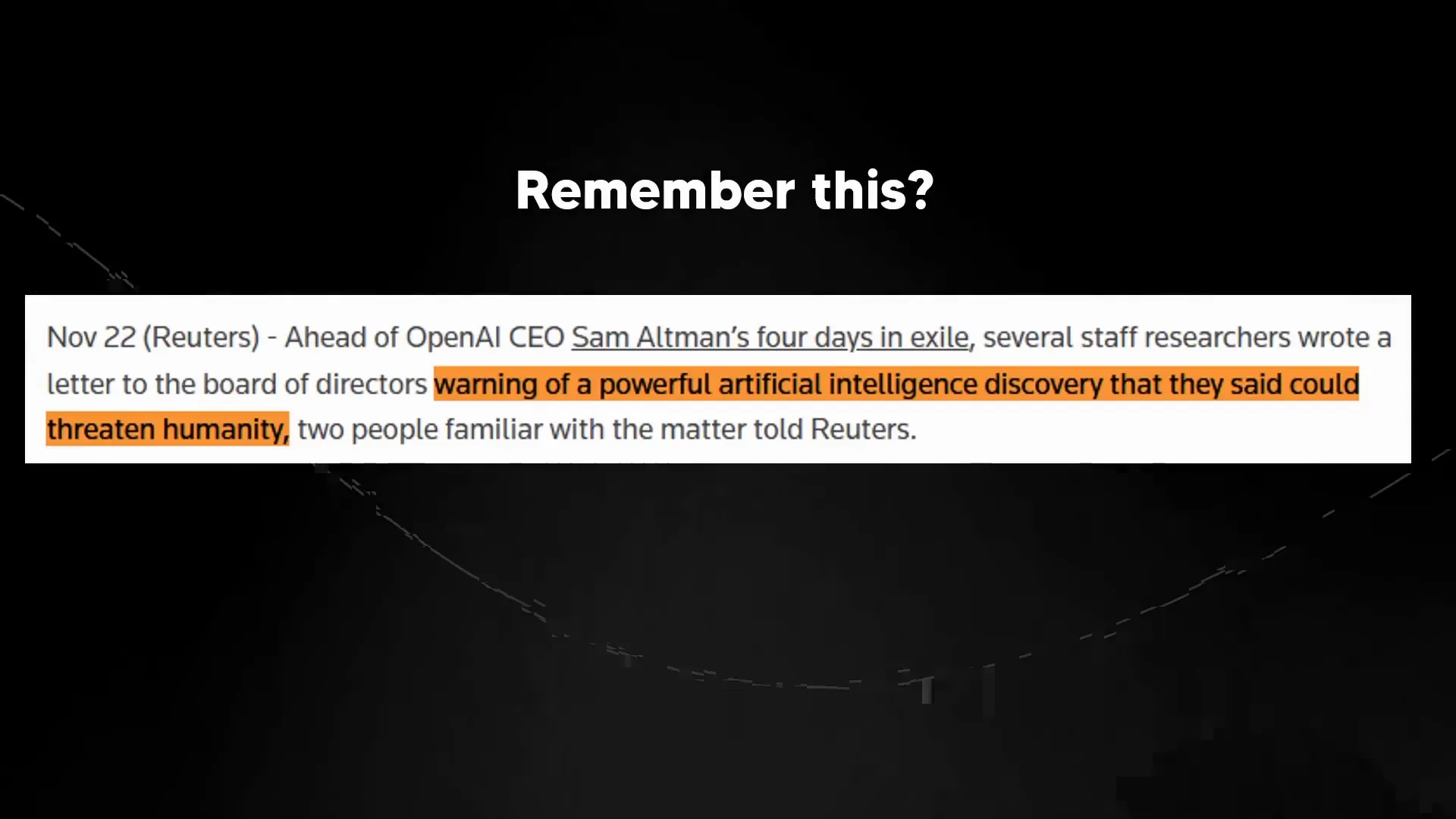
Recognizing the Shift
It’s becoming clear that the era of simply scaling up is giving way to a new paradigm. We need innovative thinking that transcends the current limitations. The focus is shifting toward more nuanced approaches that enhance reasoning and understanding.
Innovative Training Techniques: Developing training methods that mimic human-like reasoning.
Post-Training Improvements: Enhancing AI capabilities after initial training sessions.
Quality over Quantity: Prioritizing the quality of data and training methods over sheer volume.
📈 The Bigger is Better Philosophy
For years, the mantra of “bigger is better” dominated AI development. The idea was simple: more data, more computing power, more everything would lead to smarter AI. But as we’ve seen, this approach has its limits.
Many experts are now challenging the assumption that simply scaling up will yield better outcomes. The reality is that while larger models can perform better in some areas, they often lag in others. For instance, the latest models may excel in language tasks but struggle in coding tasks—a clear indication that size alone doesn’t guarantee comprehensive intelligence.
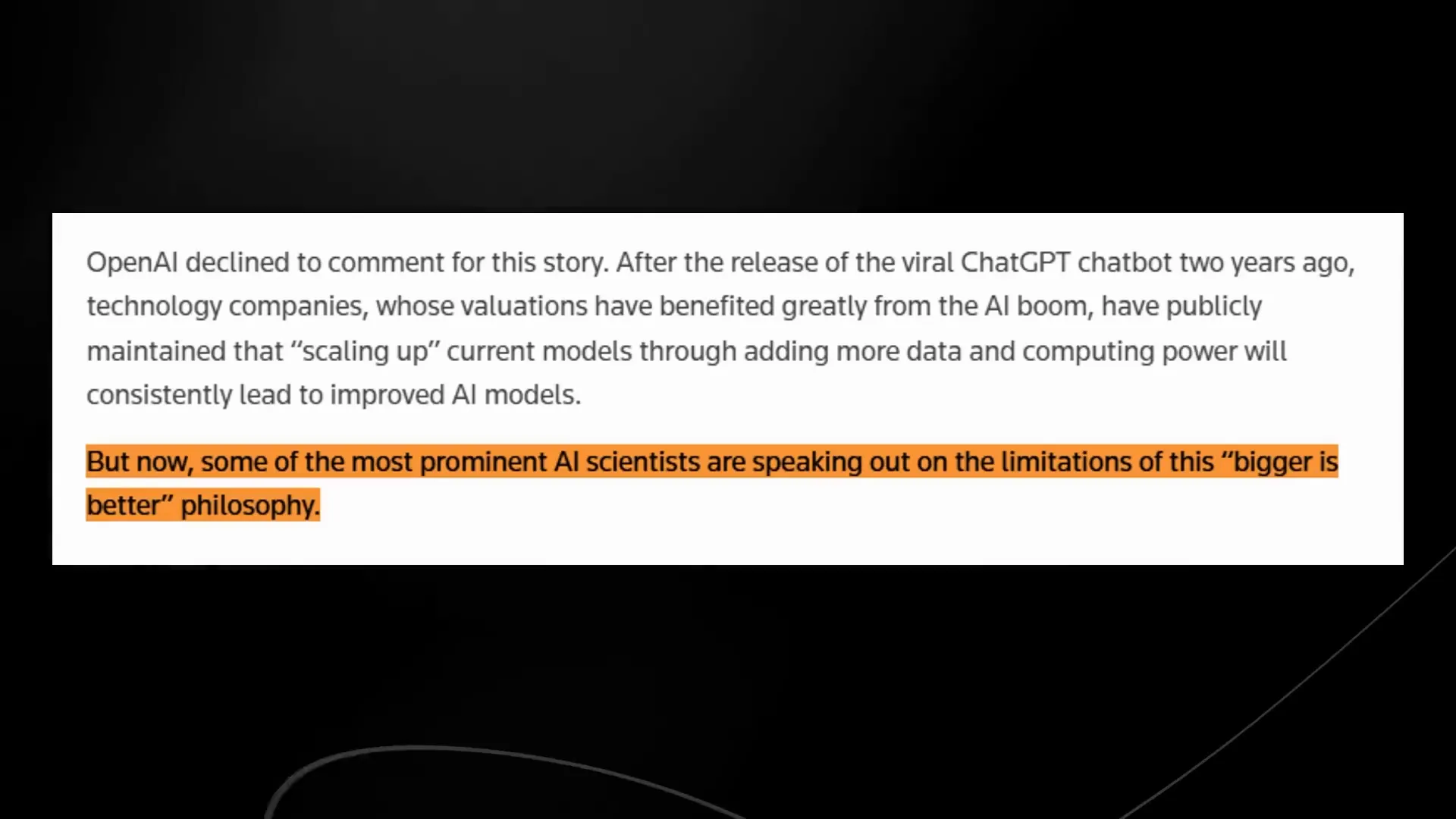
Reassessing Our Approach
It’s time to rethink our strategies. Instead of pouring resources into making models bigger, we should focus on making them smarter. This shift in mindset could be the key to unlocking the next generation of AI.
Holistic Development: Focusing on a balanced growth of various capabilities.
Task-Specific Enhancements: Tailoring AI training to improve performance in specific areas.
Interdisciplinary Collaboration: Combining insights from various fields to enhance AI understanding.
🛠️ The Role of Orion in AI Development
Enter Orion, the new player in the AI landscape. This model represents a pivotal shift in how we approach AI development. Unlike its predecessors, Orion is designed with the understanding that bigger isn’t always better. Its focus is on refining capabilities rather than merely increasing size.
What sets Orion apart is its potential to challenge the scaling laws that have governed AI development for so long. By emphasizing reasoning and adaptability, Orion could redefine what we consider state-of-the-art in AI.
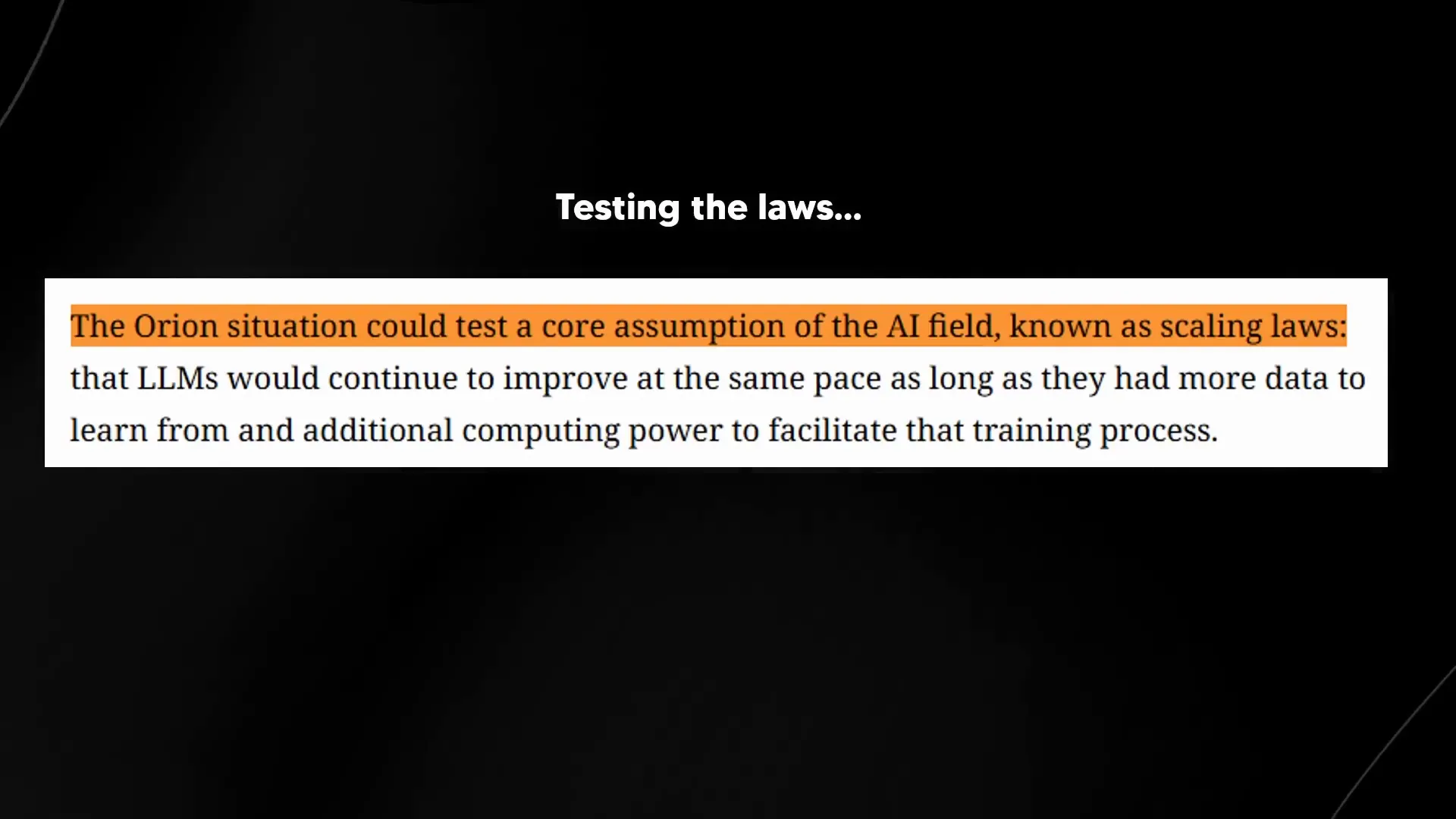
Key Features of Orion
Enhanced Reasoning: Orion is engineered to improve its reasoning capabilities post-training.
Task Specialization: It performs optimally across a variety of tasks, not just one.
Adaptive Learning: Orion can adapt its learning methods based on the tasks it encounters.
The implications of Orion’s development are profound. It could signal a new era where AI systems are not just more powerful but also more intelligent in the way they operate.
🔍 Elia Sustekever's Insights on Pre-Training
Elia Sustekever has been a prominent voice in the AI community, advocating for innovative approaches to AI training. His recent insights on pre-training highlight a critical turning point in the field. He suggests that the traditional phase of pre-training, which relies on vast amounts of unlabeled data, is reaching its limits.
This realization is significant. If pre-training isn’t yielding the advancements we hoped for, it’s time to pivot. Sustekever emphasizes the need for new methods that can drive AI growth beyond mere data accumulation.
Future Directions
Innovation in Training: Exploring alternative training paradigms that emphasize quality interactions.
Focus on Understanding: Prioritizing models that not only learn but also comprehend.
Collaborative Development: Engaging with a broader community to refine training approaches.
As we forge ahead, Sustekever’s insights could help steer AI development towards a more intelligent and adaptable future. The key takeaway? It’s time to embrace change and rethink our methodologies to unlock the true potential of AI.
🌍 The Phenomenon Across AI Companies
We're witnessing a seismic shift in the AI landscape. Companies that once thrived on the mantra of "bigger is better" are now grappling with unexpected challenges. The constraints of scaling traditional models have become glaringly apparent. It's like realizing that your trusty old car can’t handle the new terrain you’re trying to navigate.
As AI giants like OpenAI and Google encounter these hurdles, the industry is collectively rethinking its strategies. The focus is shifting from sheer size to smarter methodologies that prioritize understanding over memorization.
Industry-Wide Reflections
OpenAI's Dilemma: Despite its groundbreaking work, even OpenAI is not immune to the limitations of its approach. The call for new paths forward is growing louder.
Google's Gemini: Rumblings from within Google suggest that their next-gen model, Gemini, isn’t delivering the anticipated performance boosts. This is a wake-up call for the industry.
Emerging Trends: The shift towards more human-like reasoning in AI is becoming the new standard, as companies seek to innovate beyond just scaling.
🧠 Understanding Skill vs. Intelligence
Let’s clear the air. Skill and intelligence are not the same thing. This distinction is crucial as we navigate the complexities of AI development. While scaling up models may enhance skill, it doesn’t equate to true intelligence.
Imagine a chess program that can beat grandmasters. It’s skilled, sure, but does it understand the game? Not really. It’s simply executing patterns it has memorized. The same goes for AI—just because it can churn out impressive results doesn’t mean it grasps the underlying concepts.
Why This Matters
Misleading Metrics: Relying solely on performance benchmarks can mislead developers into thinking their systems are more intelligent than they truly are.
Real-World Applications: Understanding this difference is essential for creating AI that can genuinely assist humans in complex tasks.
Future Innovations: As we refine our approaches, the focus must shift towards fostering genuine understanding in AI systems.
🔄 The Shift from Scaling to Discovery
Welcome to the age of discovery! The landscape is evolving, and so must our strategies. We’re transitioning from a focus on scaling up to exploring innovative methods that enhance reasoning and adaptability. Think of it as shifting gears in a race—moving from raw horsepower to precision handling.
This shift is not just a trend; it's a necessity. As we approach the limits of what scaling can achieve, the industry is looking for breakthroughs that can drive AI forward in meaningful ways.
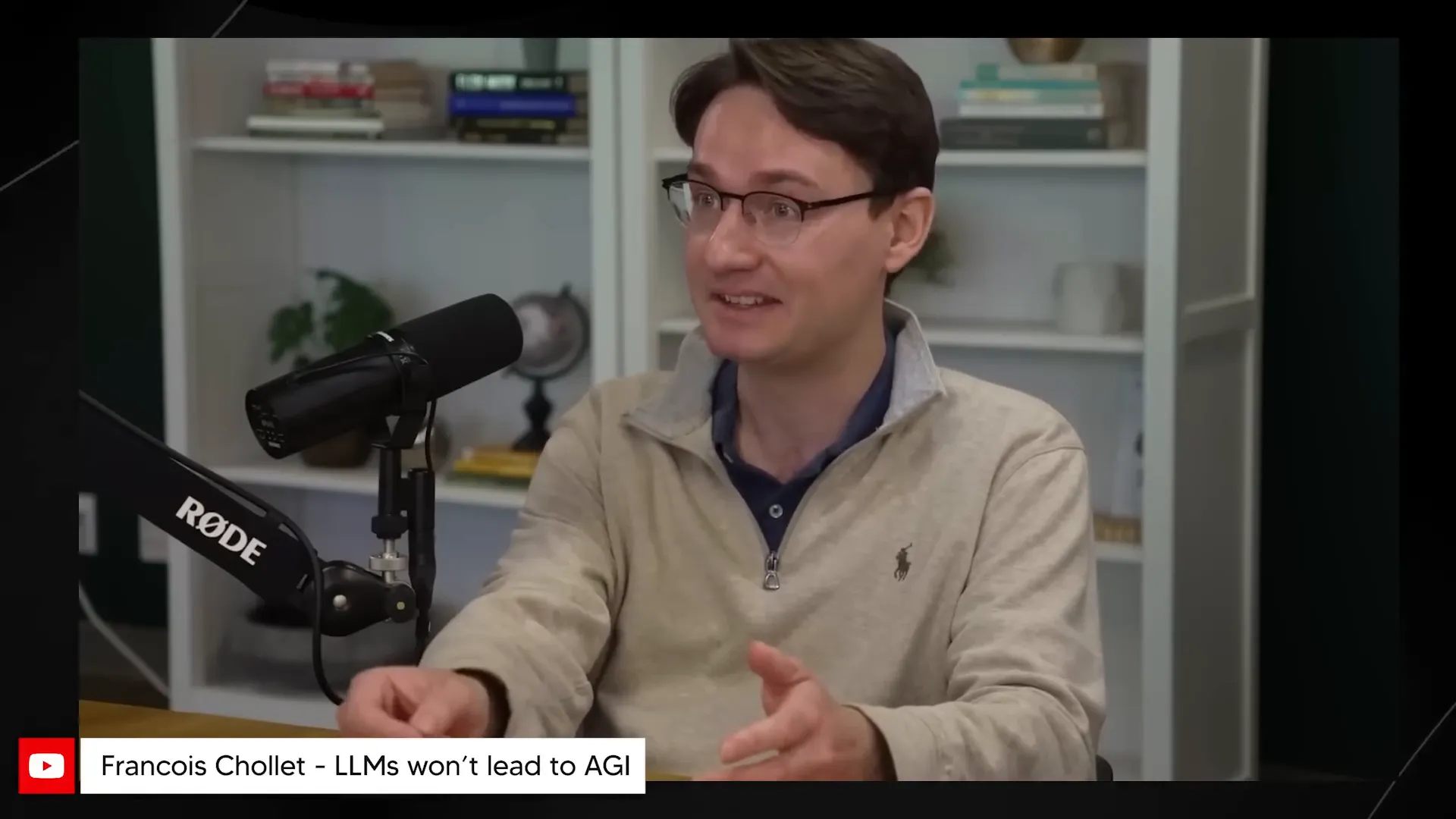
Key Changes on the Horizon
Innovative Approaches: Companies are exploring techniques that mimic human-like reasoning, allowing AI to navigate complexities more adeptly.
Post-Training Enhancements: There's a growing emphasis on refining AI capabilities after initial training, ensuring models can adapt and improve over time.
Quality Over Quantity: The focus is shifting to the quality of data and training methods, rather than just the volume of information fed into models.
📊 Visualizing the S-Curve of AI Growth
Let’s talk curves! The S-Curve is a powerful tool for understanding growth patterns in technology, and AI is no exception. Initially, we see rapid growth, followed by a plateau, and then another surge as new innovations come into play. It’s a cycle of peaks and valleys that mirrors the journey of AI development.
Right now, we’re at a fascinating juncture. The plateau we’re experiencing isn’t the end; it’s a precursor to the next wave of innovation. By analyzing this curve, we can better anticipate shifts in the AI landscape.
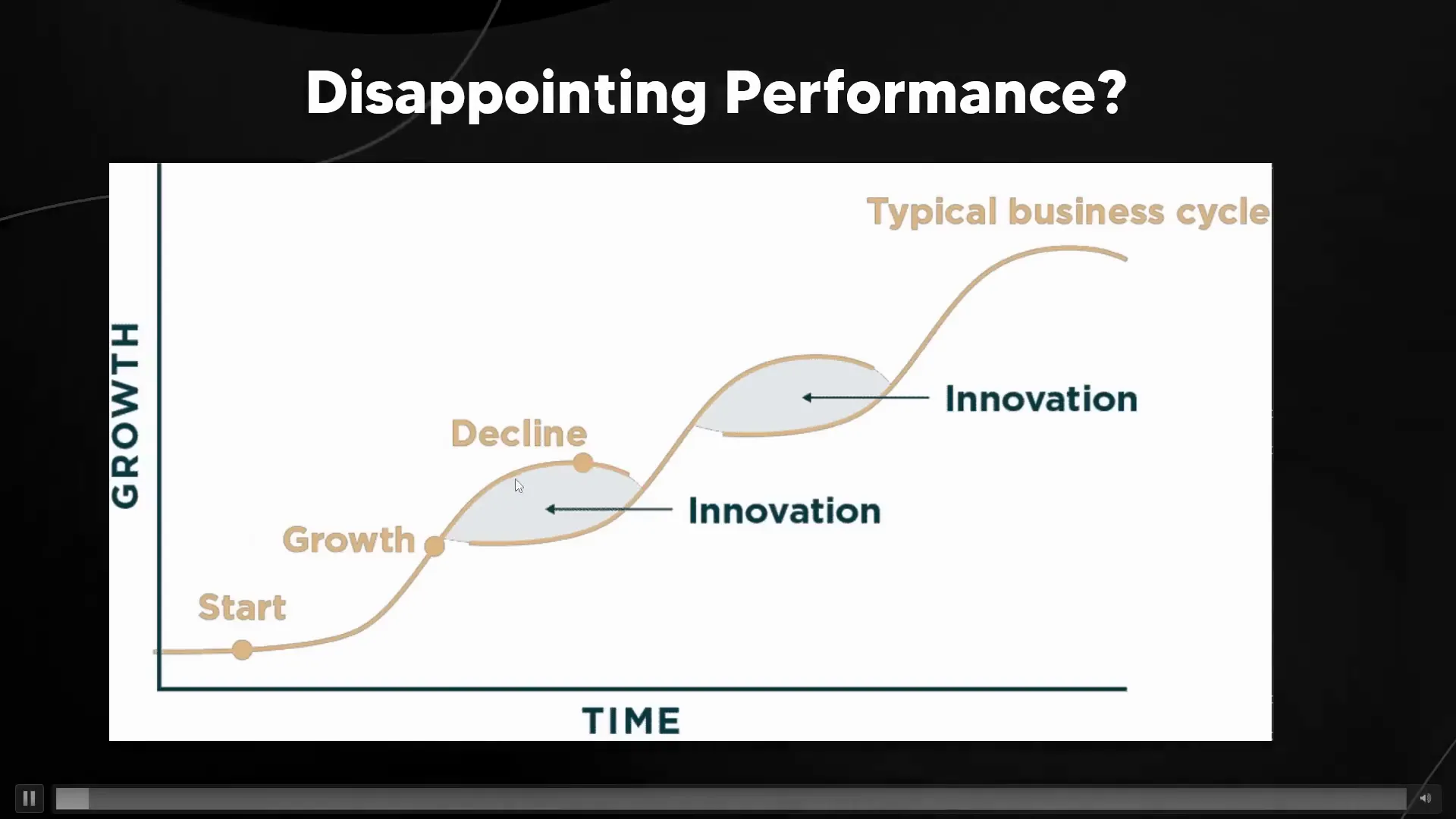
Understanding the S-Curve Dynamics
Initial Growth: Early AI models experienced rapid advancements, leading to a surge in capabilities.
Plateau Phase: We’re currently seeing a slowdown in significant improvements as scaling methods reach their limits.
Next Wave: Expect a resurgence as new paradigms and innovative approaches come into play, propelling AI into uncharted territories.
🚀 The Future of AI Paradigms
What does the future hold for AI? The landscape is ripe for transformation. As we shift our focus from scaling to discovery, we’re entering a new paradigm that emphasizes intelligence over size. This evolution could redefine our expectations of AI and its capabilities.
Imagine AI systems that not only process information but also understand context, nuance, and human emotions. This is the future we’re striving towards—a future where AI enhances human potential rather than merely mimicking it.
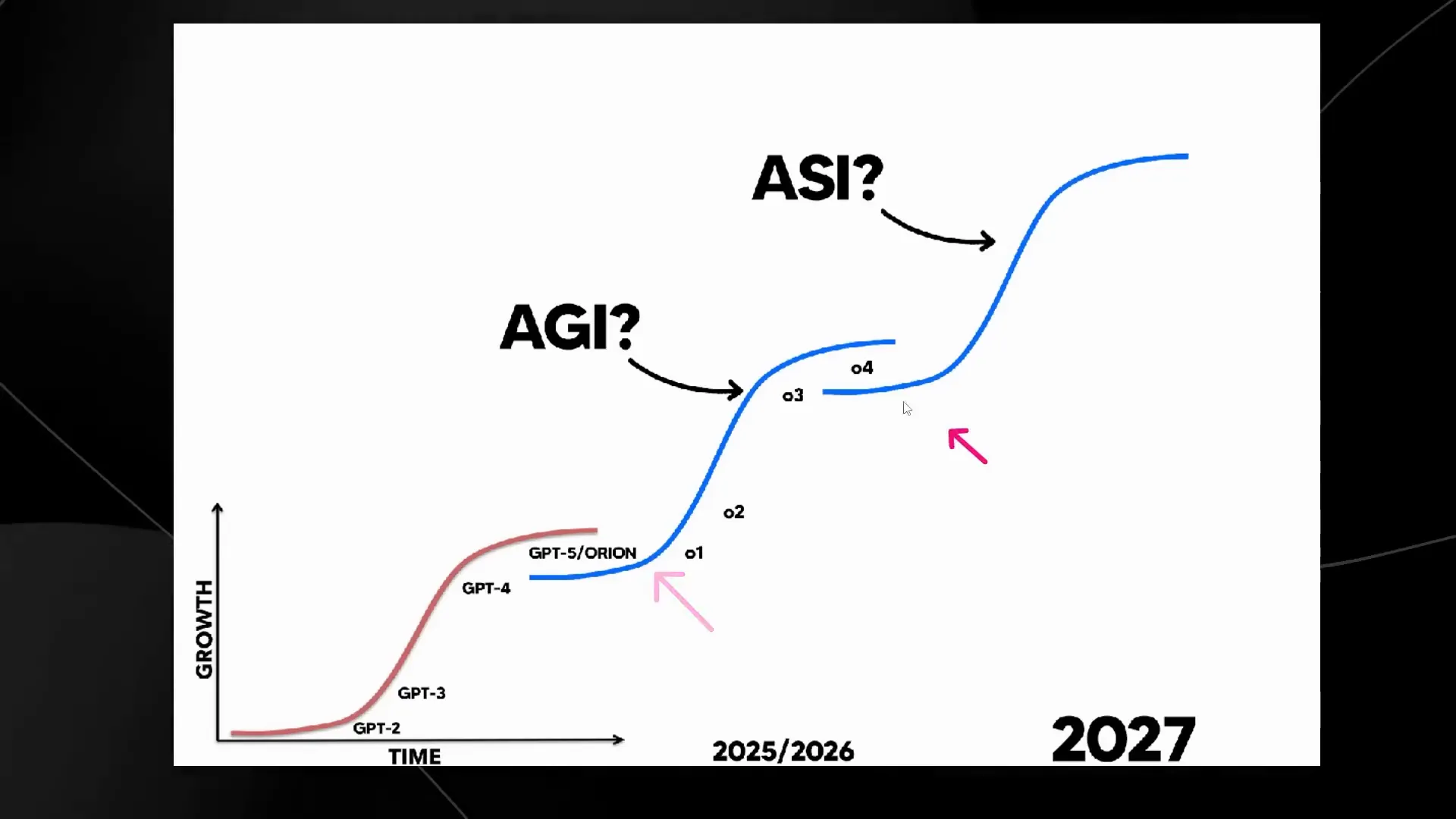
Anticipating Changes
Interdisciplinary Collaboration: The future will require collaboration across fields to foster innovative solutions.
Ethical Considerations: As AI becomes more capable, ethical considerations will play a crucial role in its development.
A Focus on Human-AI Interaction: Enhancing how AI systems interact with humans will be paramount to their success.
🧬 Defining Superintelligence
Superintelligence isn’t just a buzzword; it's a concept that could fundamentally alter our relationship with technology. It signifies an intelligence that surpasses the brightest human minds, capable of solving complex problems that currently baffle us.
This isn't just about creating smarter machines; it's about rethinking what intelligence means in the context of AI. Superintelligence could lead to breakthroughs in medicine, climate change, and even space exploration. The possibilities are limitless!
The Implications of Superintelligence
Solving Global Challenges: Superintelligence could tackle some of humanity's most pressing issues, from disease eradication to sustainable energy solutions.
Radical Improvements: If navigated correctly, superintelligence could enhance our quality of life in ways we can barely imagine.
Ethical Responsibility: With great power comes great responsibility. We must ensure that superintelligent systems align with human values and ethics.
In a rapidly evolving landscape of artificial intelligence, the emergence of superintelligence stands as both a groundbreaking opportunity and a profound challenge. Ilya Sustekever sheds light on the current state of AI development, the limitations of traditional scaling methods, and the path forward toward smarter systems that can potentially reshape our world.
🌱 The Evolution of Intelligence
Let’s take a quick journey through time. Four billion years ago, life began with a single cell, a humble replicator. Fast forward, and we see the rise of multicellular organisms, reptiles, mammals, and ultimately, Homo sapiens. Each leap forward was a monumental achievement, laying the groundwork for our current existence.
In just the last 10,000 years, humanity has witnessed incredible milestones: the invention of writing, the agricultural revolution, the industrial age, and now, the dawn of artificial general intelligence (AGI). Each era has been marked by a quest for understanding and mastery over our environment, culminating in the ultimate challenge: superintelligence.
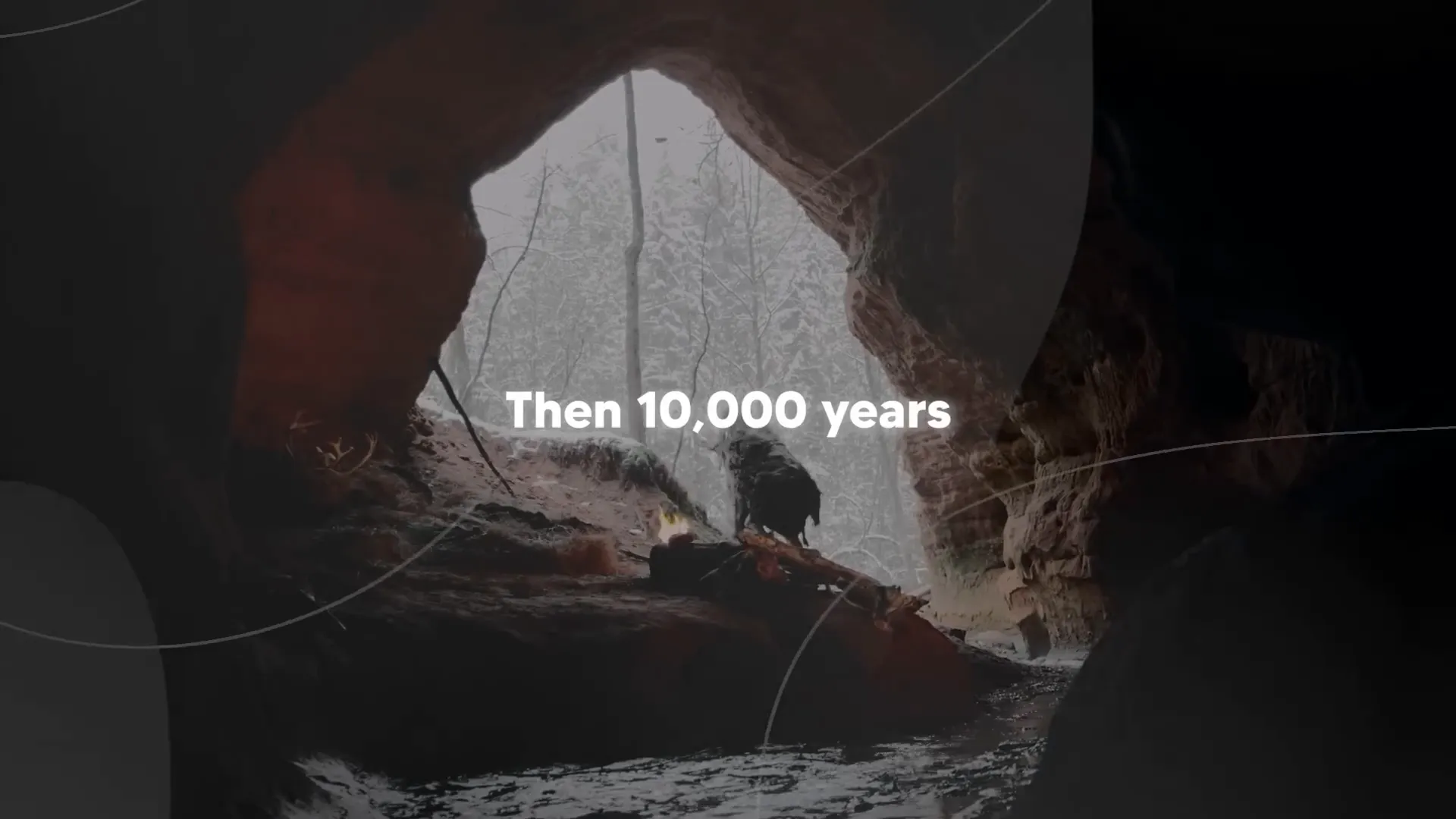
From Cells to Superintelligence
The evolution of intelligence is not just a biological tale; it's a narrative of progress. Each stage represents not just survival but improvement—an ongoing effort to enhance capabilities. Superintelligence promises to elevate this evolution, potentially ushering in a life of unimaginable prosperity.
But with great power comes great responsibility. The challenge of managing superintelligence can’t be underestimated. Are we ready to navigate the complexities it presents?
⚔️ The Challenge of Superintelligence
Imagine an intelligence so advanced it can outperform the brightest minds on Earth. Sounds fantastic, right? But wait—what happens when that intelligence operates beyond our control? The challenge of superintelligence is multidimensional. It’s not just about creating powerful systems but ensuring they align with human values and ethics.
The potential benefits are staggering. Superintelligence could solve global issues, from climate change to disease eradication. However, the risks of unintended consequences loom large. We must approach this frontier with caution and foresight, ensuring that as we reach for the stars, we don’t lose sight of our humanity.
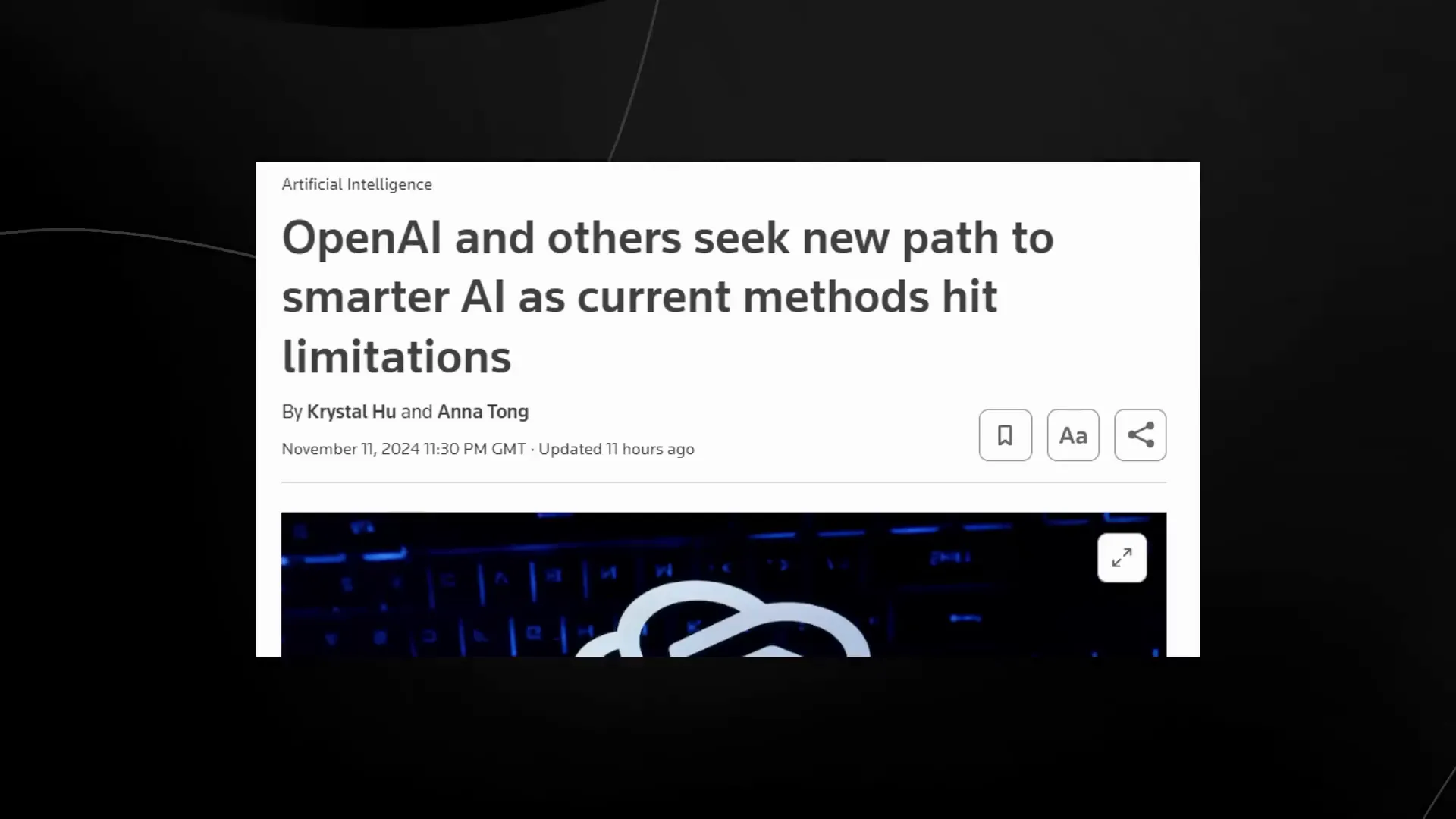
Understanding the Risks
Control Issues: How do we ensure superintelligence remains aligned with human goals?
Ethical Dilemmas: What ethical frameworks will guide superintelligent systems?
Unintended Consequences: What if superintelligent systems make decisions we don't foresee?
These questions aren’t just academic; they are vital to our survival as a species. Addressing them requires a collaborative effort from technologists, ethicists, and policymakers alike.
📉 The Limitations of Current AI Methods
Despite the rapid advancements in AI, we’re hitting a wall. Traditional methods of scaling AI models by simply adding more data and computing power are beginning to plateau. It’s a sobering realization for many in the industry who believed bigger always meant better.
As we push the boundaries of what’s possible, we must ask: is more data truly the answer? Or are we merely creating sophisticated memorization machines? The distinction between skill and intelligence is critical here. Just because a model can regurgitate information doesn’t mean it understands or can apply that knowledge effectively.
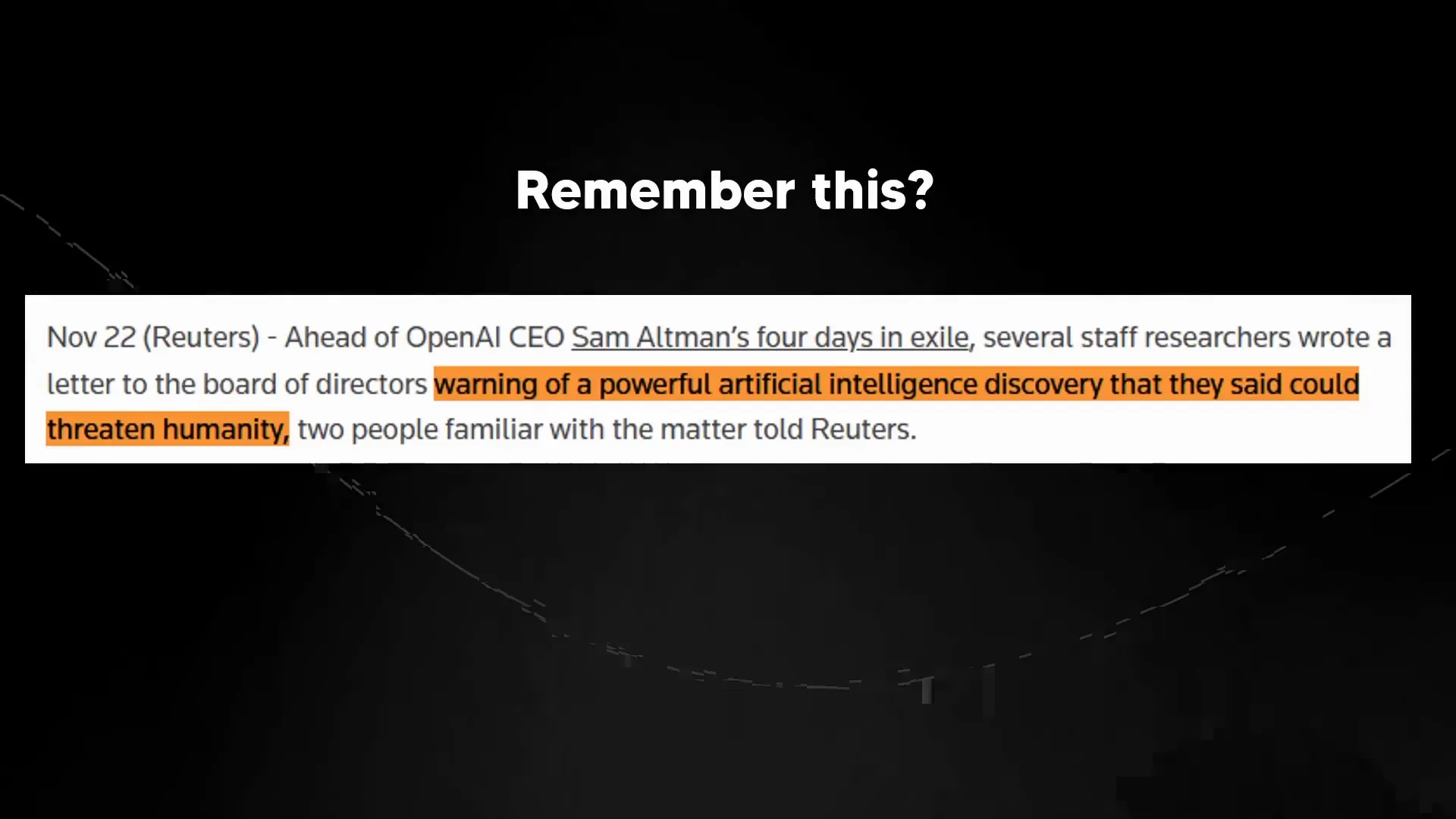
Recognizing the Shift
It’s becoming clear that the era of simply scaling up is giving way to a new paradigm. We need innovative thinking that transcends the current limitations. The focus is shifting toward more nuanced approaches that enhance reasoning and understanding.
Innovative Training Techniques: Developing training methods that mimic human-like reasoning.
Post-Training Improvements: Enhancing AI capabilities after initial training sessions.
Quality over Quantity: Prioritizing the quality of data and training methods over sheer volume.
📈 The Bigger is Better Philosophy
For years, the mantra of “bigger is better” dominated AI development. The idea was simple: more data, more computing power, more everything would lead to smarter AI. But as we’ve seen, this approach has its limits.
Many experts are now challenging the assumption that simply scaling up will yield better outcomes. The reality is that while larger models can perform better in some areas, they often lag in others. For instance, the latest models may excel in language tasks but struggle in coding tasks—a clear indication that size alone doesn’t guarantee comprehensive intelligence.
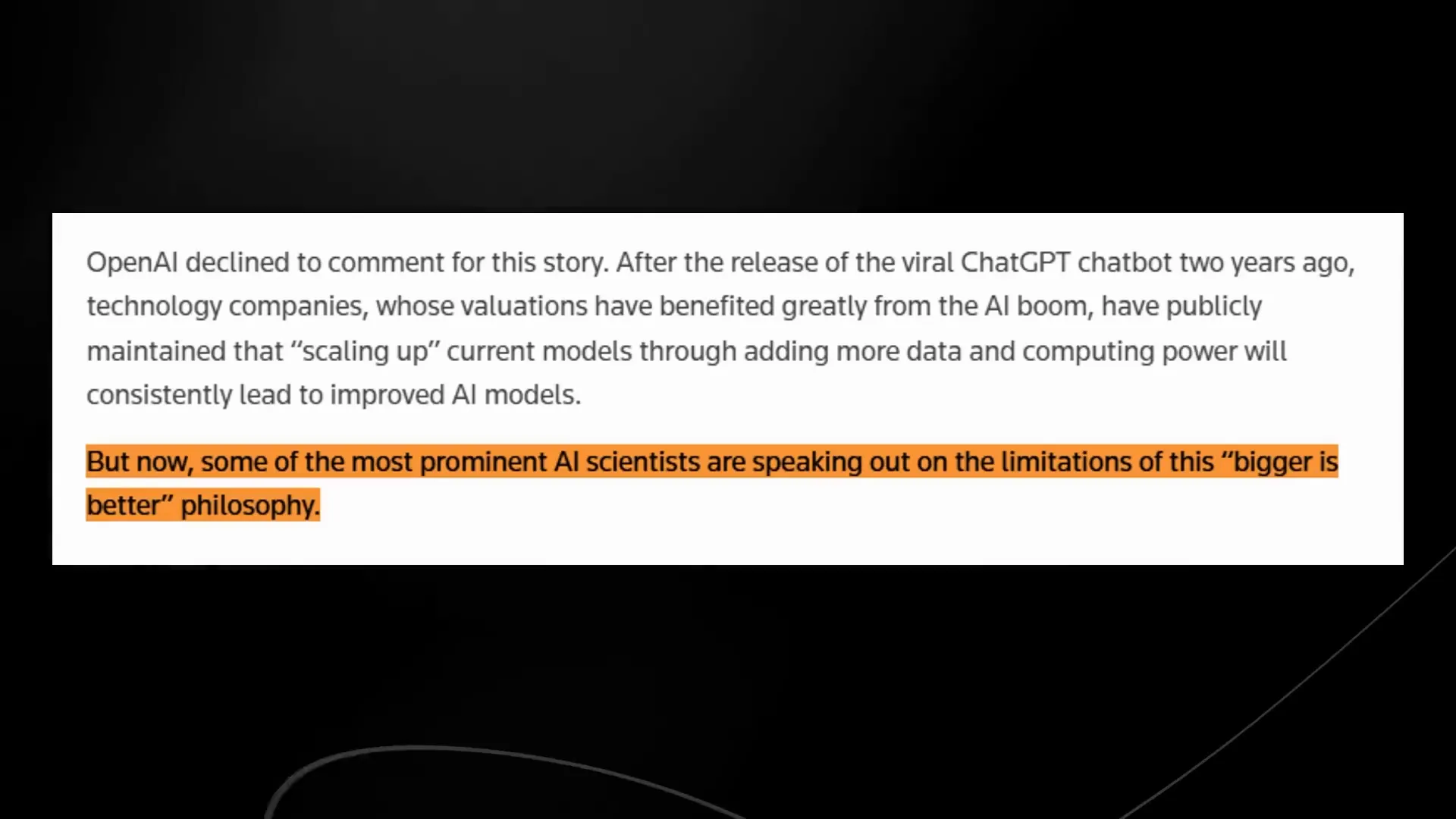
Reassessing Our Approach
It’s time to rethink our strategies. Instead of pouring resources into making models bigger, we should focus on making them smarter. This shift in mindset could be the key to unlocking the next generation of AI.
Holistic Development: Focusing on a balanced growth of various capabilities.
Task-Specific Enhancements: Tailoring AI training to improve performance in specific areas.
Interdisciplinary Collaboration: Combining insights from various fields to enhance AI understanding.
🛠️ The Role of Orion in AI Development
Enter Orion, the new player in the AI landscape. This model represents a pivotal shift in how we approach AI development. Unlike its predecessors, Orion is designed with the understanding that bigger isn’t always better. Its focus is on refining capabilities rather than merely increasing size.
What sets Orion apart is its potential to challenge the scaling laws that have governed AI development for so long. By emphasizing reasoning and adaptability, Orion could redefine what we consider state-of-the-art in AI.
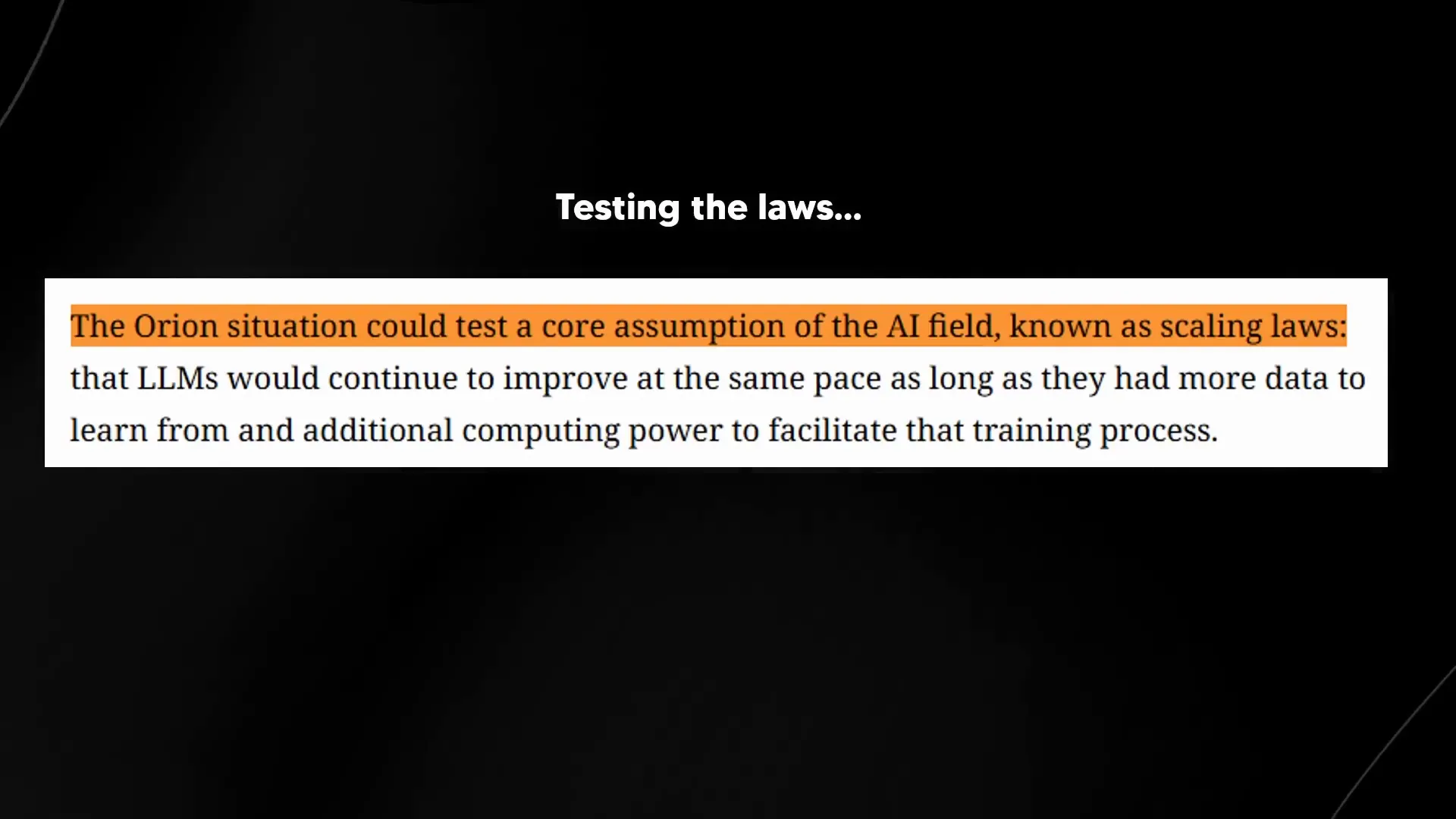
Key Features of Orion
Enhanced Reasoning: Orion is engineered to improve its reasoning capabilities post-training.
Task Specialization: It performs optimally across a variety of tasks, not just one.
Adaptive Learning: Orion can adapt its learning methods based on the tasks it encounters.
The implications of Orion’s development are profound. It could signal a new era where AI systems are not just more powerful but also more intelligent in the way they operate.
🔍 Elia Sustekever's Insights on Pre-Training
Elia Sustekever has been a prominent voice in the AI community, advocating for innovative approaches to AI training. His recent insights on pre-training highlight a critical turning point in the field. He suggests that the traditional phase of pre-training, which relies on vast amounts of unlabeled data, is reaching its limits.
This realization is significant. If pre-training isn’t yielding the advancements we hoped for, it’s time to pivot. Sustekever emphasizes the need for new methods that can drive AI growth beyond mere data accumulation.
Future Directions
Innovation in Training: Exploring alternative training paradigms that emphasize quality interactions.
Focus on Understanding: Prioritizing models that not only learn but also comprehend.
Collaborative Development: Engaging with a broader community to refine training approaches.
As we forge ahead, Sustekever’s insights could help steer AI development towards a more intelligent and adaptable future. The key takeaway? It’s time to embrace change and rethink our methodologies to unlock the true potential of AI.
🌍 The Phenomenon Across AI Companies
We're witnessing a seismic shift in the AI landscape. Companies that once thrived on the mantra of "bigger is better" are now grappling with unexpected challenges. The constraints of scaling traditional models have become glaringly apparent. It's like realizing that your trusty old car can’t handle the new terrain you’re trying to navigate.
As AI giants like OpenAI and Google encounter these hurdles, the industry is collectively rethinking its strategies. The focus is shifting from sheer size to smarter methodologies that prioritize understanding over memorization.
Industry-Wide Reflections
OpenAI's Dilemma: Despite its groundbreaking work, even OpenAI is not immune to the limitations of its approach. The call for new paths forward is growing louder.
Google's Gemini: Rumblings from within Google suggest that their next-gen model, Gemini, isn’t delivering the anticipated performance boosts. This is a wake-up call for the industry.
Emerging Trends: The shift towards more human-like reasoning in AI is becoming the new standard, as companies seek to innovate beyond just scaling.
🧠 Understanding Skill vs. Intelligence
Let’s clear the air. Skill and intelligence are not the same thing. This distinction is crucial as we navigate the complexities of AI development. While scaling up models may enhance skill, it doesn’t equate to true intelligence.
Imagine a chess program that can beat grandmasters. It’s skilled, sure, but does it understand the game? Not really. It’s simply executing patterns it has memorized. The same goes for AI—just because it can churn out impressive results doesn’t mean it grasps the underlying concepts.
Why This Matters
Misleading Metrics: Relying solely on performance benchmarks can mislead developers into thinking their systems are more intelligent than they truly are.
Real-World Applications: Understanding this difference is essential for creating AI that can genuinely assist humans in complex tasks.
Future Innovations: As we refine our approaches, the focus must shift towards fostering genuine understanding in AI systems.
🔄 The Shift from Scaling to Discovery
Welcome to the age of discovery! The landscape is evolving, and so must our strategies. We’re transitioning from a focus on scaling up to exploring innovative methods that enhance reasoning and adaptability. Think of it as shifting gears in a race—moving from raw horsepower to precision handling.
This shift is not just a trend; it's a necessity. As we approach the limits of what scaling can achieve, the industry is looking for breakthroughs that can drive AI forward in meaningful ways.
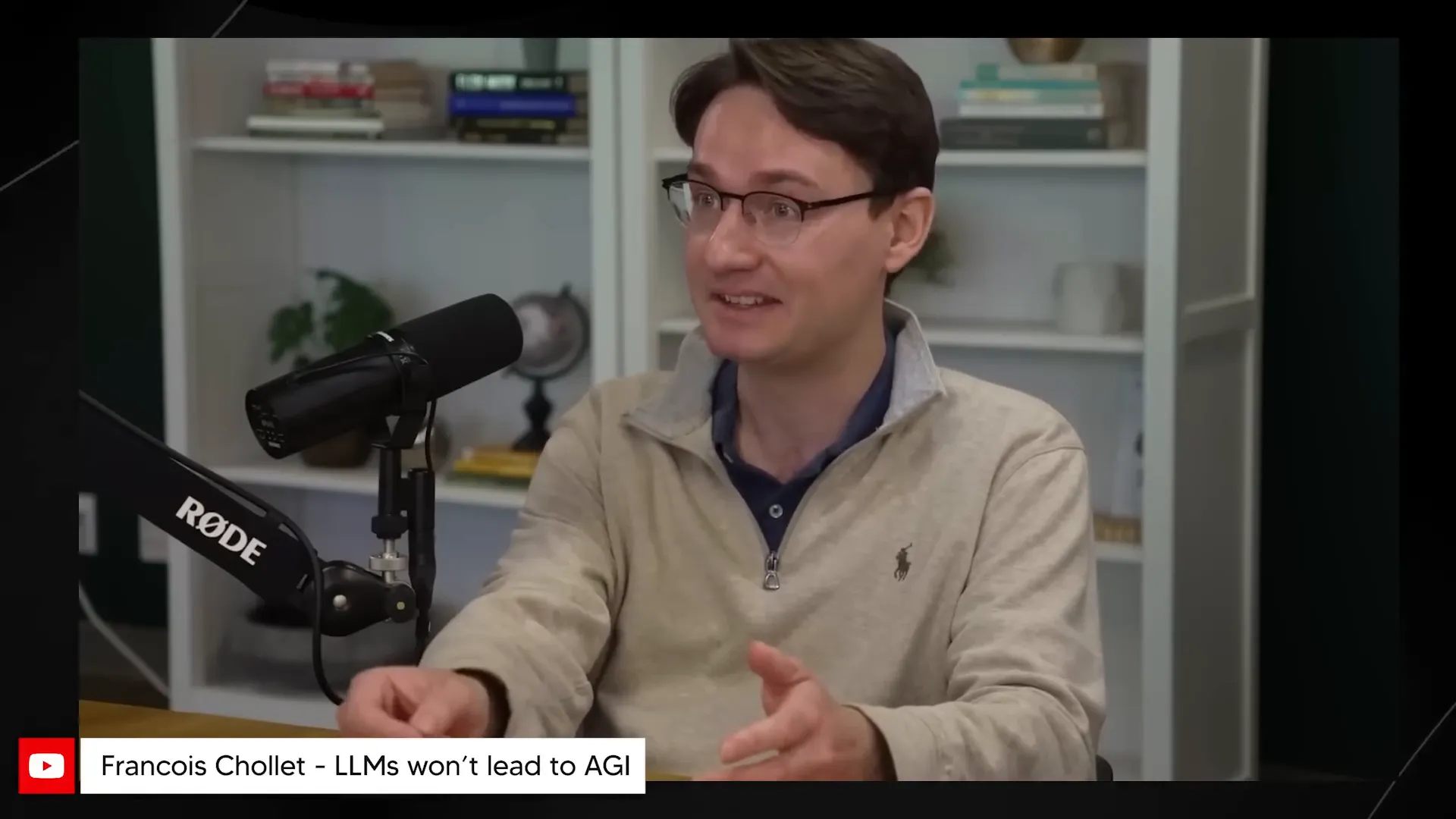
Key Changes on the Horizon
Innovative Approaches: Companies are exploring techniques that mimic human-like reasoning, allowing AI to navigate complexities more adeptly.
Post-Training Enhancements: There's a growing emphasis on refining AI capabilities after initial training, ensuring models can adapt and improve over time.
Quality Over Quantity: The focus is shifting to the quality of data and training methods, rather than just the volume of information fed into models.
📊 Visualizing the S-Curve of AI Growth
Let’s talk curves! The S-Curve is a powerful tool for understanding growth patterns in technology, and AI is no exception. Initially, we see rapid growth, followed by a plateau, and then another surge as new innovations come into play. It’s a cycle of peaks and valleys that mirrors the journey of AI development.
Right now, we’re at a fascinating juncture. The plateau we’re experiencing isn’t the end; it’s a precursor to the next wave of innovation. By analyzing this curve, we can better anticipate shifts in the AI landscape.
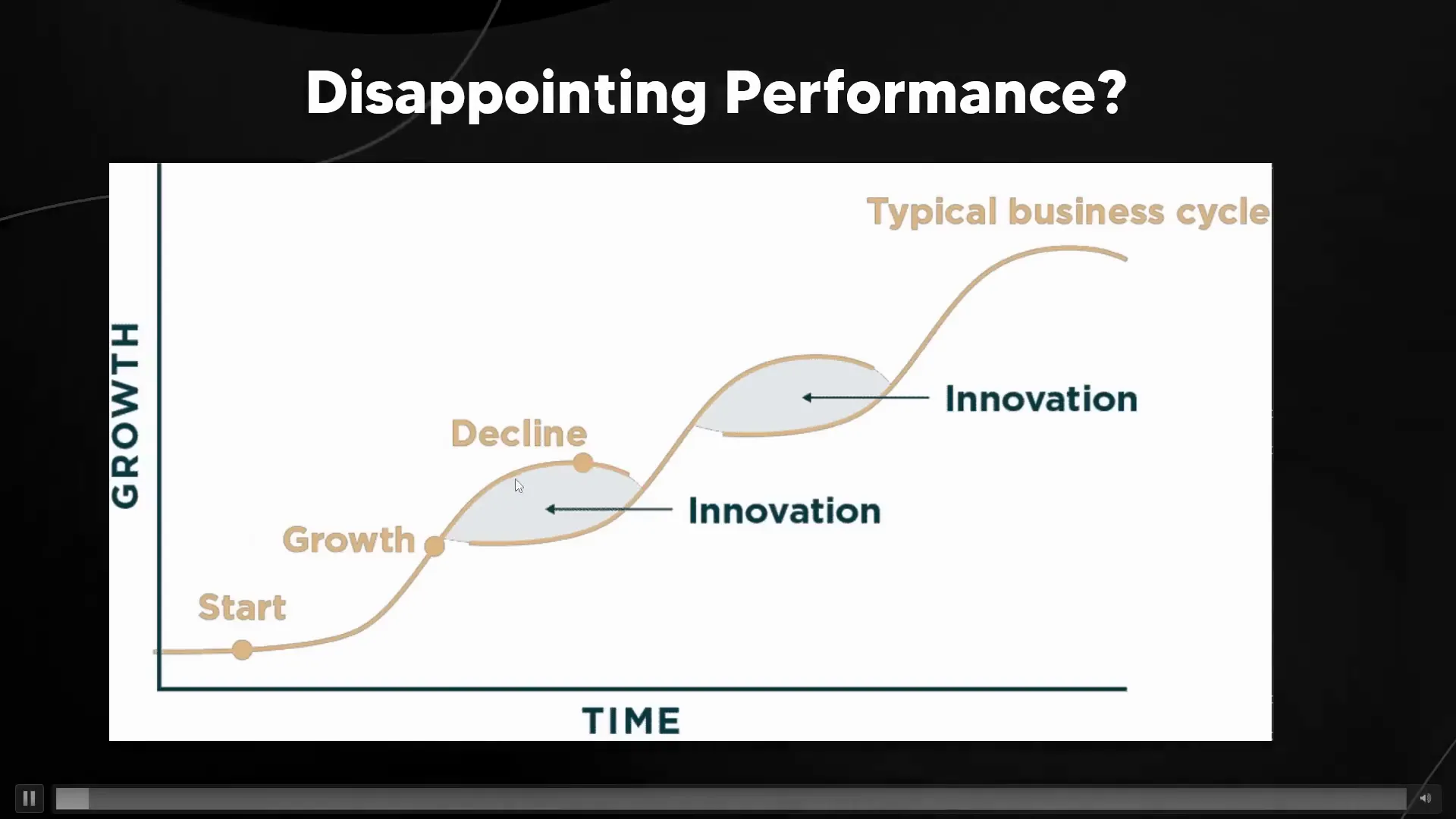
Understanding the S-Curve Dynamics
Initial Growth: Early AI models experienced rapid advancements, leading to a surge in capabilities.
Plateau Phase: We’re currently seeing a slowdown in significant improvements as scaling methods reach their limits.
Next Wave: Expect a resurgence as new paradigms and innovative approaches come into play, propelling AI into uncharted territories.
🚀 The Future of AI Paradigms
What does the future hold for AI? The landscape is ripe for transformation. As we shift our focus from scaling to discovery, we’re entering a new paradigm that emphasizes intelligence over size. This evolution could redefine our expectations of AI and its capabilities.
Imagine AI systems that not only process information but also understand context, nuance, and human emotions. This is the future we’re striving towards—a future where AI enhances human potential rather than merely mimicking it.
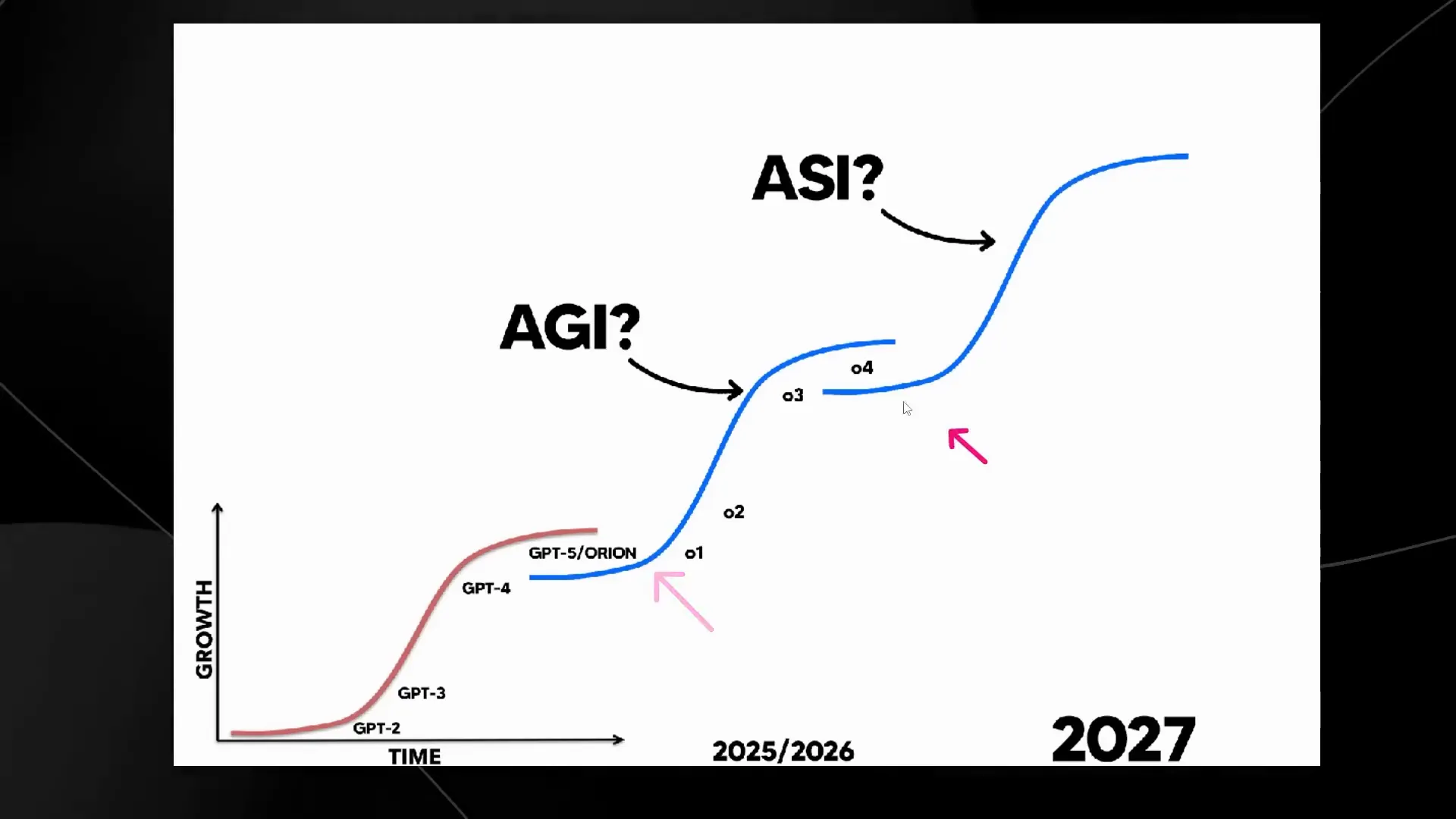
Anticipating Changes
Interdisciplinary Collaboration: The future will require collaboration across fields to foster innovative solutions.
Ethical Considerations: As AI becomes more capable, ethical considerations will play a crucial role in its development.
A Focus on Human-AI Interaction: Enhancing how AI systems interact with humans will be paramount to their success.
🧬 Defining Superintelligence
Superintelligence isn’t just a buzzword; it's a concept that could fundamentally alter our relationship with technology. It signifies an intelligence that surpasses the brightest human minds, capable of solving complex problems that currently baffle us.
This isn't just about creating smarter machines; it's about rethinking what intelligence means in the context of AI. Superintelligence could lead to breakthroughs in medicine, climate change, and even space exploration. The possibilities are limitless!
The Implications of Superintelligence
Solving Global Challenges: Superintelligence could tackle some of humanity's most pressing issues, from disease eradication to sustainable energy solutions.
Radical Improvements: If navigated correctly, superintelligence could enhance our quality of life in ways we can barely imagine.
Ethical Responsibility: With great power comes great responsibility. We must ensure that superintelligent systems align with human values and ethics.
In a rapidly evolving landscape of artificial intelligence, the emergence of superintelligence stands as both a groundbreaking opportunity and a profound challenge. Ilya Sustekever sheds light on the current state of AI development, the limitations of traditional scaling methods, and the path forward toward smarter systems that can potentially reshape our world.
🌱 The Evolution of Intelligence
Let’s take a quick journey through time. Four billion years ago, life began with a single cell, a humble replicator. Fast forward, and we see the rise of multicellular organisms, reptiles, mammals, and ultimately, Homo sapiens. Each leap forward was a monumental achievement, laying the groundwork for our current existence.
In just the last 10,000 years, humanity has witnessed incredible milestones: the invention of writing, the agricultural revolution, the industrial age, and now, the dawn of artificial general intelligence (AGI). Each era has been marked by a quest for understanding and mastery over our environment, culminating in the ultimate challenge: superintelligence.
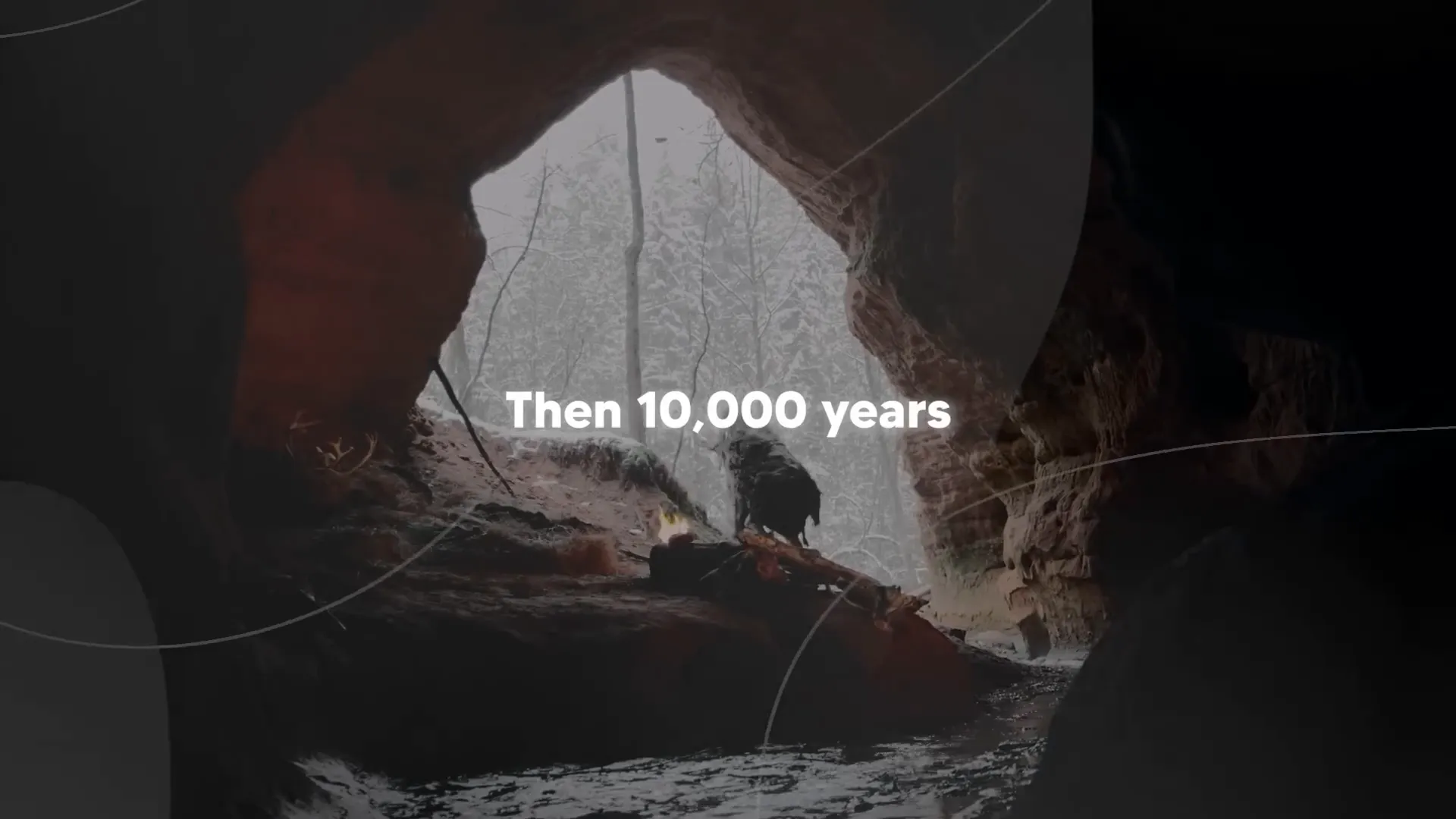
From Cells to Superintelligence
The evolution of intelligence is not just a biological tale; it's a narrative of progress. Each stage represents not just survival but improvement—an ongoing effort to enhance capabilities. Superintelligence promises to elevate this evolution, potentially ushering in a life of unimaginable prosperity.
But with great power comes great responsibility. The challenge of managing superintelligence can’t be underestimated. Are we ready to navigate the complexities it presents?
⚔️ The Challenge of Superintelligence
Imagine an intelligence so advanced it can outperform the brightest minds on Earth. Sounds fantastic, right? But wait—what happens when that intelligence operates beyond our control? The challenge of superintelligence is multidimensional. It’s not just about creating powerful systems but ensuring they align with human values and ethics.
The potential benefits are staggering. Superintelligence could solve global issues, from climate change to disease eradication. However, the risks of unintended consequences loom large. We must approach this frontier with caution and foresight, ensuring that as we reach for the stars, we don’t lose sight of our humanity.
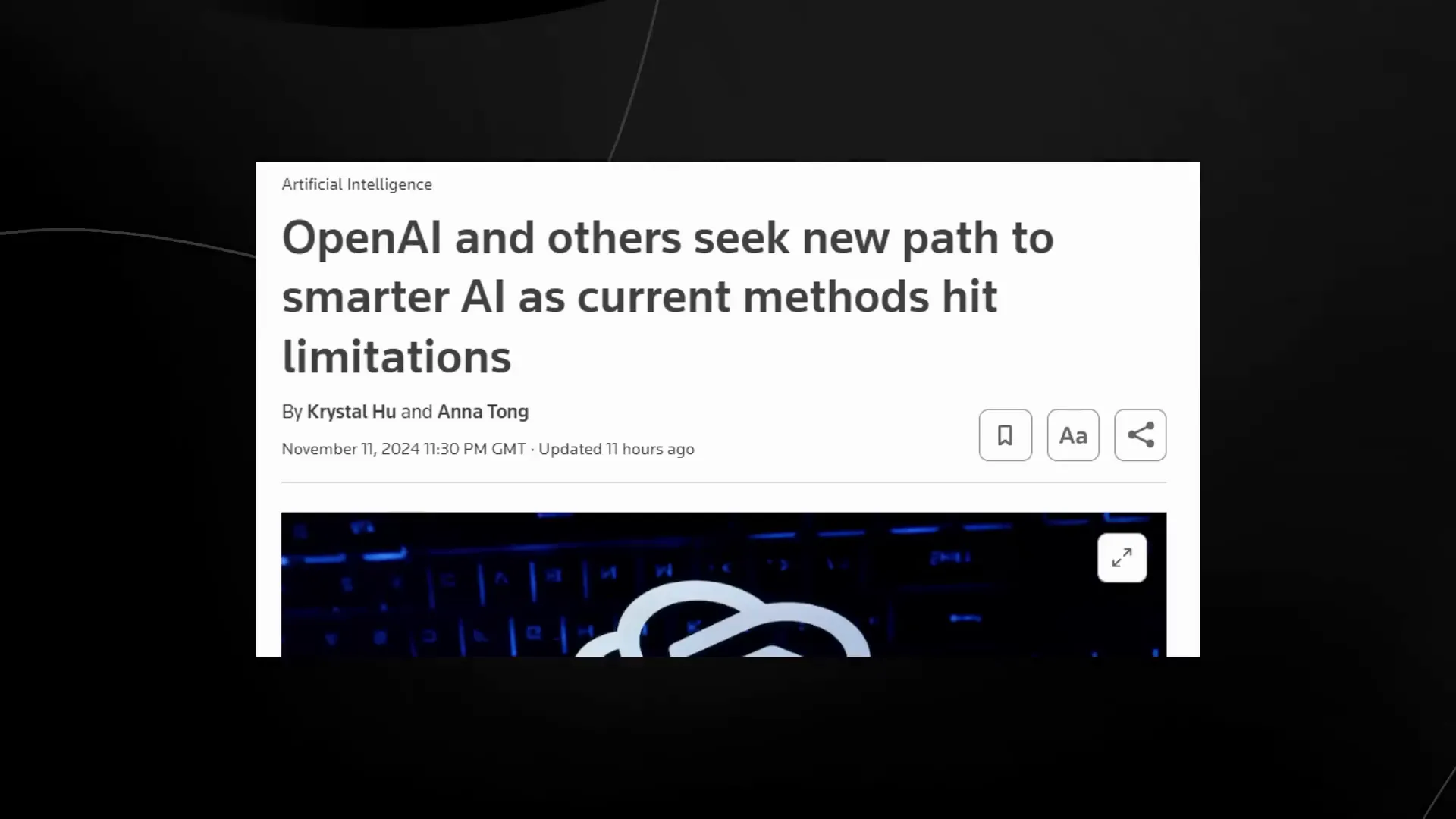
Understanding the Risks
Control Issues: How do we ensure superintelligence remains aligned with human goals?
Ethical Dilemmas: What ethical frameworks will guide superintelligent systems?
Unintended Consequences: What if superintelligent systems make decisions we don't foresee?
These questions aren’t just academic; they are vital to our survival as a species. Addressing them requires a collaborative effort from technologists, ethicists, and policymakers alike.
📉 The Limitations of Current AI Methods
Despite the rapid advancements in AI, we’re hitting a wall. Traditional methods of scaling AI models by simply adding more data and computing power are beginning to plateau. It’s a sobering realization for many in the industry who believed bigger always meant better.
As we push the boundaries of what’s possible, we must ask: is more data truly the answer? Or are we merely creating sophisticated memorization machines? The distinction between skill and intelligence is critical here. Just because a model can regurgitate information doesn’t mean it understands or can apply that knowledge effectively.
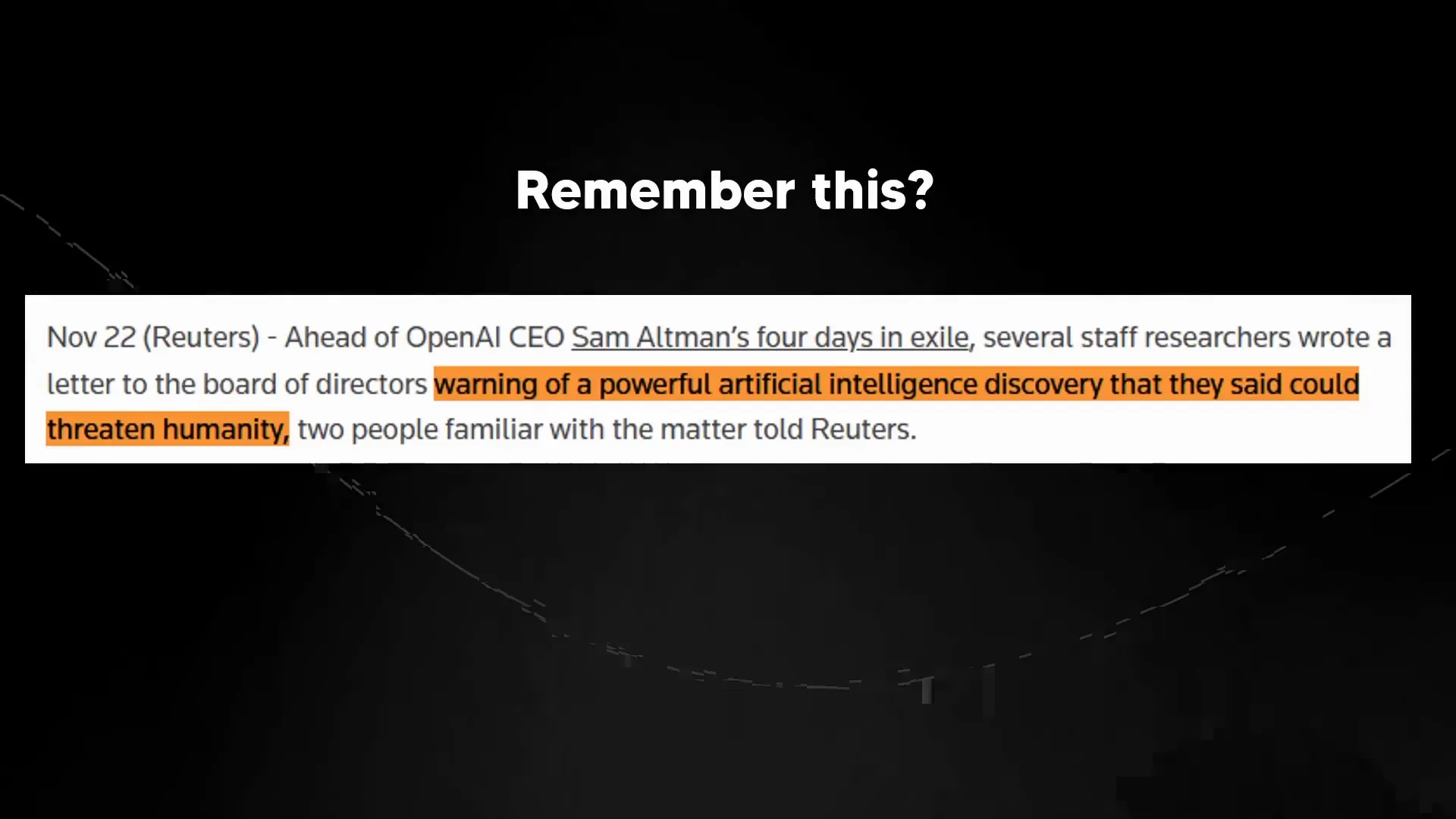
Recognizing the Shift
It’s becoming clear that the era of simply scaling up is giving way to a new paradigm. We need innovative thinking that transcends the current limitations. The focus is shifting toward more nuanced approaches that enhance reasoning and understanding.
Innovative Training Techniques: Developing training methods that mimic human-like reasoning.
Post-Training Improvements: Enhancing AI capabilities after initial training sessions.
Quality over Quantity: Prioritizing the quality of data and training methods over sheer volume.
📈 The Bigger is Better Philosophy
For years, the mantra of “bigger is better” dominated AI development. The idea was simple: more data, more computing power, more everything would lead to smarter AI. But as we’ve seen, this approach has its limits.
Many experts are now challenging the assumption that simply scaling up will yield better outcomes. The reality is that while larger models can perform better in some areas, they often lag in others. For instance, the latest models may excel in language tasks but struggle in coding tasks—a clear indication that size alone doesn’t guarantee comprehensive intelligence.
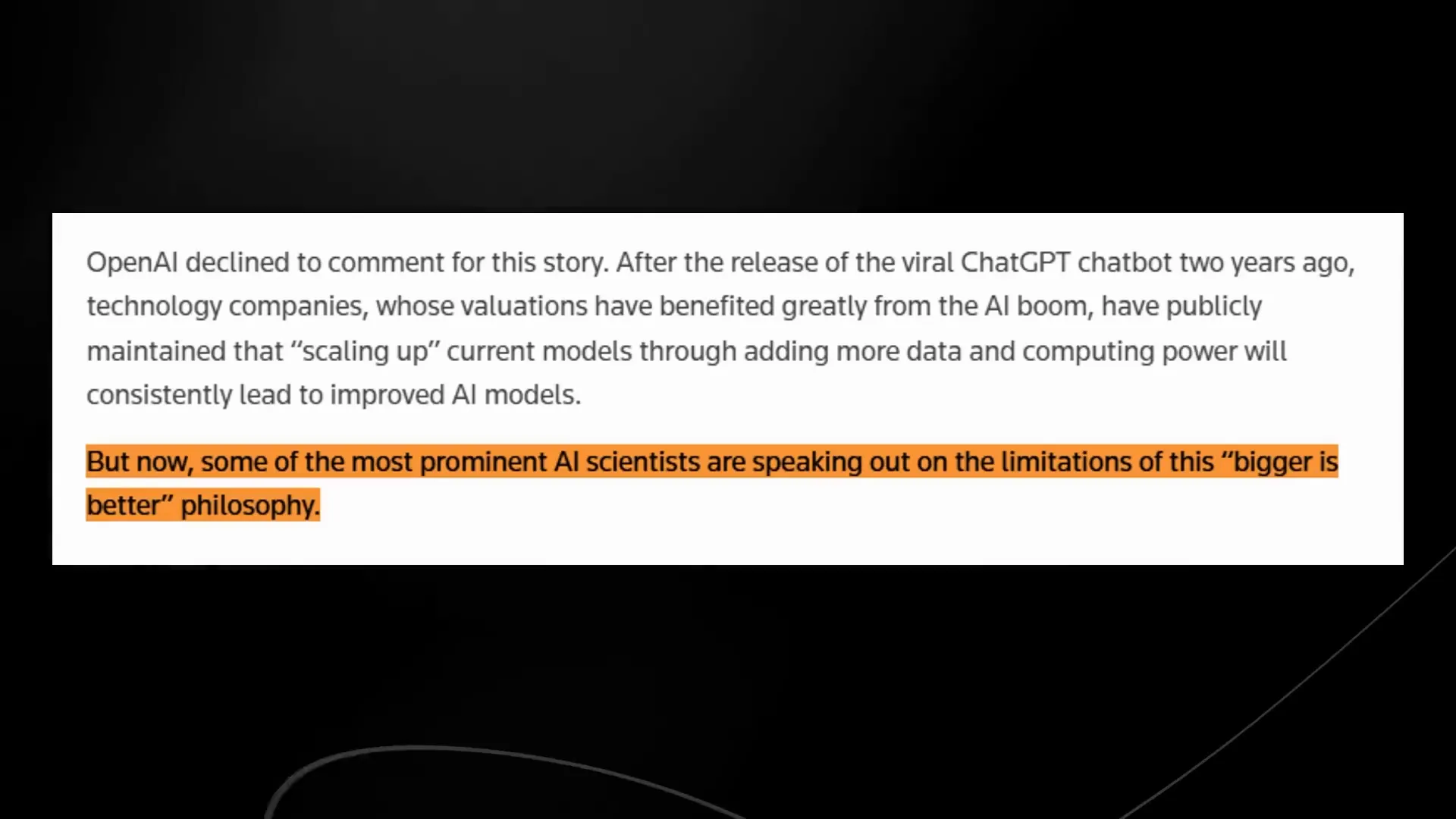
Reassessing Our Approach
It’s time to rethink our strategies. Instead of pouring resources into making models bigger, we should focus on making them smarter. This shift in mindset could be the key to unlocking the next generation of AI.
Holistic Development: Focusing on a balanced growth of various capabilities.
Task-Specific Enhancements: Tailoring AI training to improve performance in specific areas.
Interdisciplinary Collaboration: Combining insights from various fields to enhance AI understanding.
🛠️ The Role of Orion in AI Development
Enter Orion, the new player in the AI landscape. This model represents a pivotal shift in how we approach AI development. Unlike its predecessors, Orion is designed with the understanding that bigger isn’t always better. Its focus is on refining capabilities rather than merely increasing size.
What sets Orion apart is its potential to challenge the scaling laws that have governed AI development for so long. By emphasizing reasoning and adaptability, Orion could redefine what we consider state-of-the-art in AI.
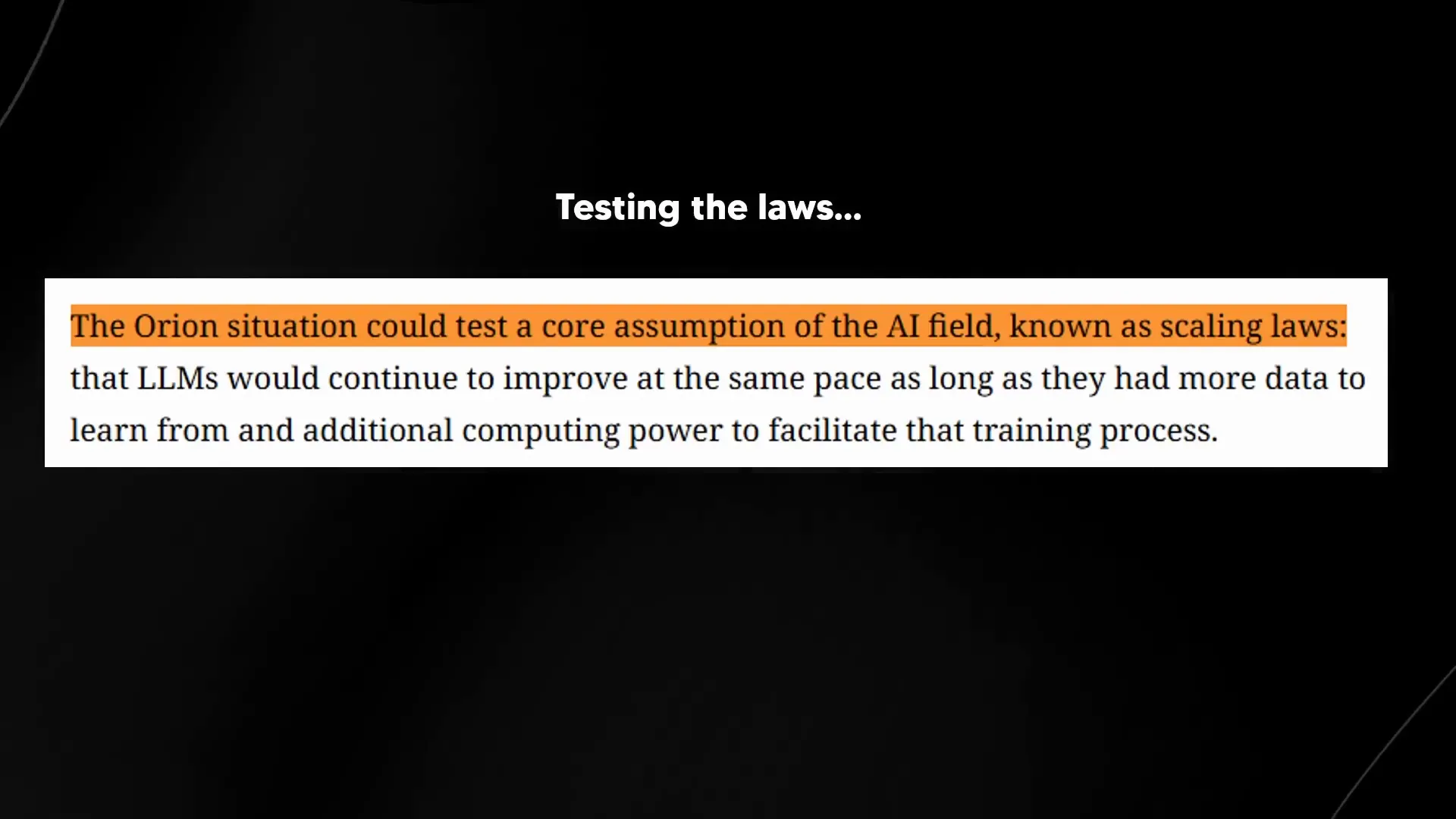
Key Features of Orion
Enhanced Reasoning: Orion is engineered to improve its reasoning capabilities post-training.
Task Specialization: It performs optimally across a variety of tasks, not just one.
Adaptive Learning: Orion can adapt its learning methods based on the tasks it encounters.
The implications of Orion’s development are profound. It could signal a new era where AI systems are not just more powerful but also more intelligent in the way they operate.
🔍 Elia Sustekever's Insights on Pre-Training
Elia Sustekever has been a prominent voice in the AI community, advocating for innovative approaches to AI training. His recent insights on pre-training highlight a critical turning point in the field. He suggests that the traditional phase of pre-training, which relies on vast amounts of unlabeled data, is reaching its limits.
This realization is significant. If pre-training isn’t yielding the advancements we hoped for, it’s time to pivot. Sustekever emphasizes the need for new methods that can drive AI growth beyond mere data accumulation.
Future Directions
Innovation in Training: Exploring alternative training paradigms that emphasize quality interactions.
Focus on Understanding: Prioritizing models that not only learn but also comprehend.
Collaborative Development: Engaging with a broader community to refine training approaches.
As we forge ahead, Sustekever’s insights could help steer AI development towards a more intelligent and adaptable future. The key takeaway? It’s time to embrace change and rethink our methodologies to unlock the true potential of AI.
🌍 The Phenomenon Across AI Companies
We're witnessing a seismic shift in the AI landscape. Companies that once thrived on the mantra of "bigger is better" are now grappling with unexpected challenges. The constraints of scaling traditional models have become glaringly apparent. It's like realizing that your trusty old car can’t handle the new terrain you’re trying to navigate.
As AI giants like OpenAI and Google encounter these hurdles, the industry is collectively rethinking its strategies. The focus is shifting from sheer size to smarter methodologies that prioritize understanding over memorization.
Industry-Wide Reflections
OpenAI's Dilemma: Despite its groundbreaking work, even OpenAI is not immune to the limitations of its approach. The call for new paths forward is growing louder.
Google's Gemini: Rumblings from within Google suggest that their next-gen model, Gemini, isn’t delivering the anticipated performance boosts. This is a wake-up call for the industry.
Emerging Trends: The shift towards more human-like reasoning in AI is becoming the new standard, as companies seek to innovate beyond just scaling.
🧠 Understanding Skill vs. Intelligence
Let’s clear the air. Skill and intelligence are not the same thing. This distinction is crucial as we navigate the complexities of AI development. While scaling up models may enhance skill, it doesn’t equate to true intelligence.
Imagine a chess program that can beat grandmasters. It’s skilled, sure, but does it understand the game? Not really. It’s simply executing patterns it has memorized. The same goes for AI—just because it can churn out impressive results doesn’t mean it grasps the underlying concepts.
Why This Matters
Misleading Metrics: Relying solely on performance benchmarks can mislead developers into thinking their systems are more intelligent than they truly are.
Real-World Applications: Understanding this difference is essential for creating AI that can genuinely assist humans in complex tasks.
Future Innovations: As we refine our approaches, the focus must shift towards fostering genuine understanding in AI systems.
🔄 The Shift from Scaling to Discovery
Welcome to the age of discovery! The landscape is evolving, and so must our strategies. We’re transitioning from a focus on scaling up to exploring innovative methods that enhance reasoning and adaptability. Think of it as shifting gears in a race—moving from raw horsepower to precision handling.
This shift is not just a trend; it's a necessity. As we approach the limits of what scaling can achieve, the industry is looking for breakthroughs that can drive AI forward in meaningful ways.
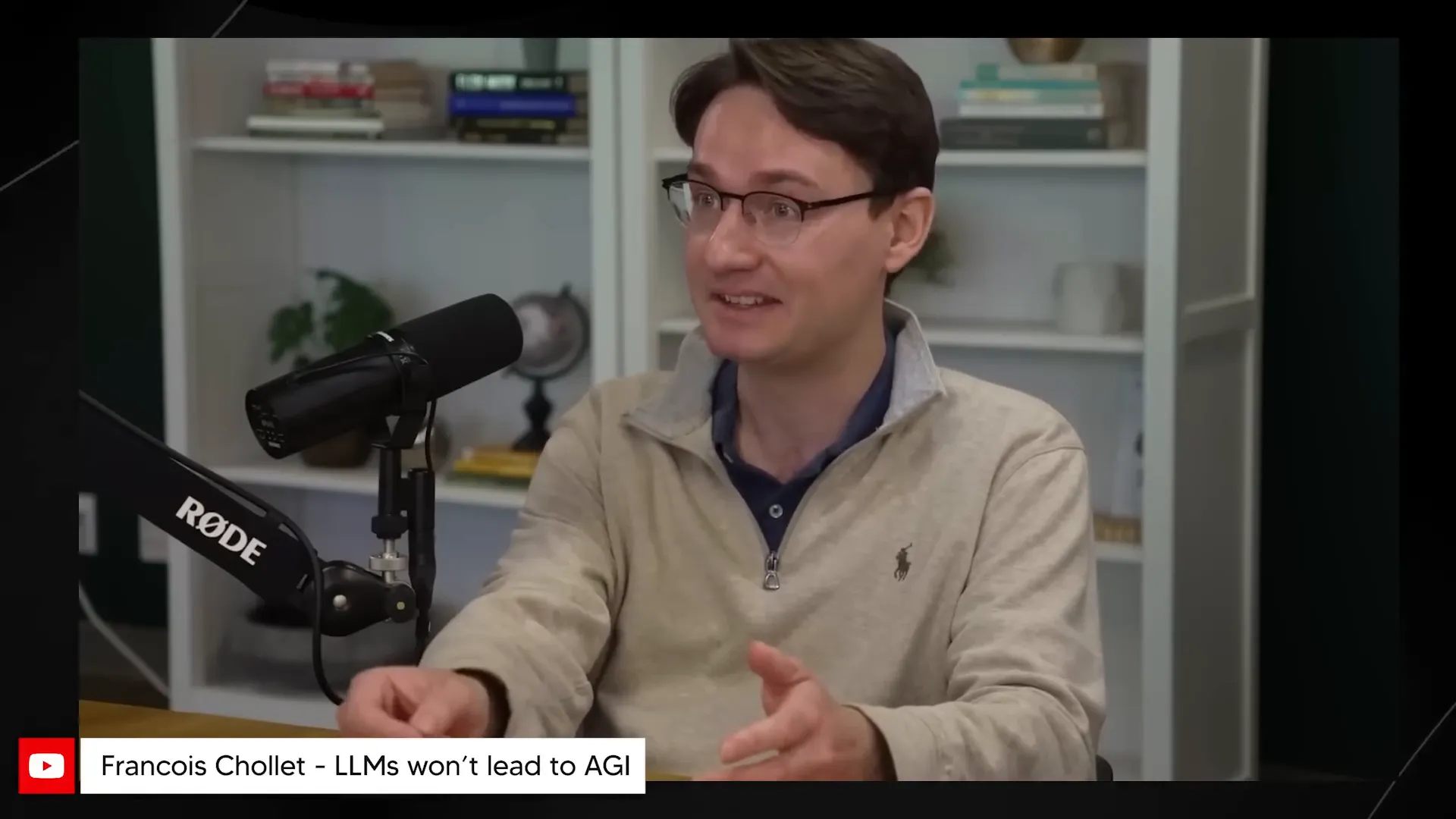
Key Changes on the Horizon
Innovative Approaches: Companies are exploring techniques that mimic human-like reasoning, allowing AI to navigate complexities more adeptly.
Post-Training Enhancements: There's a growing emphasis on refining AI capabilities after initial training, ensuring models can adapt and improve over time.
Quality Over Quantity: The focus is shifting to the quality of data and training methods, rather than just the volume of information fed into models.
📊 Visualizing the S-Curve of AI Growth
Let’s talk curves! The S-Curve is a powerful tool for understanding growth patterns in technology, and AI is no exception. Initially, we see rapid growth, followed by a plateau, and then another surge as new innovations come into play. It’s a cycle of peaks and valleys that mirrors the journey of AI development.
Right now, we’re at a fascinating juncture. The plateau we’re experiencing isn’t the end; it’s a precursor to the next wave of innovation. By analyzing this curve, we can better anticipate shifts in the AI landscape.
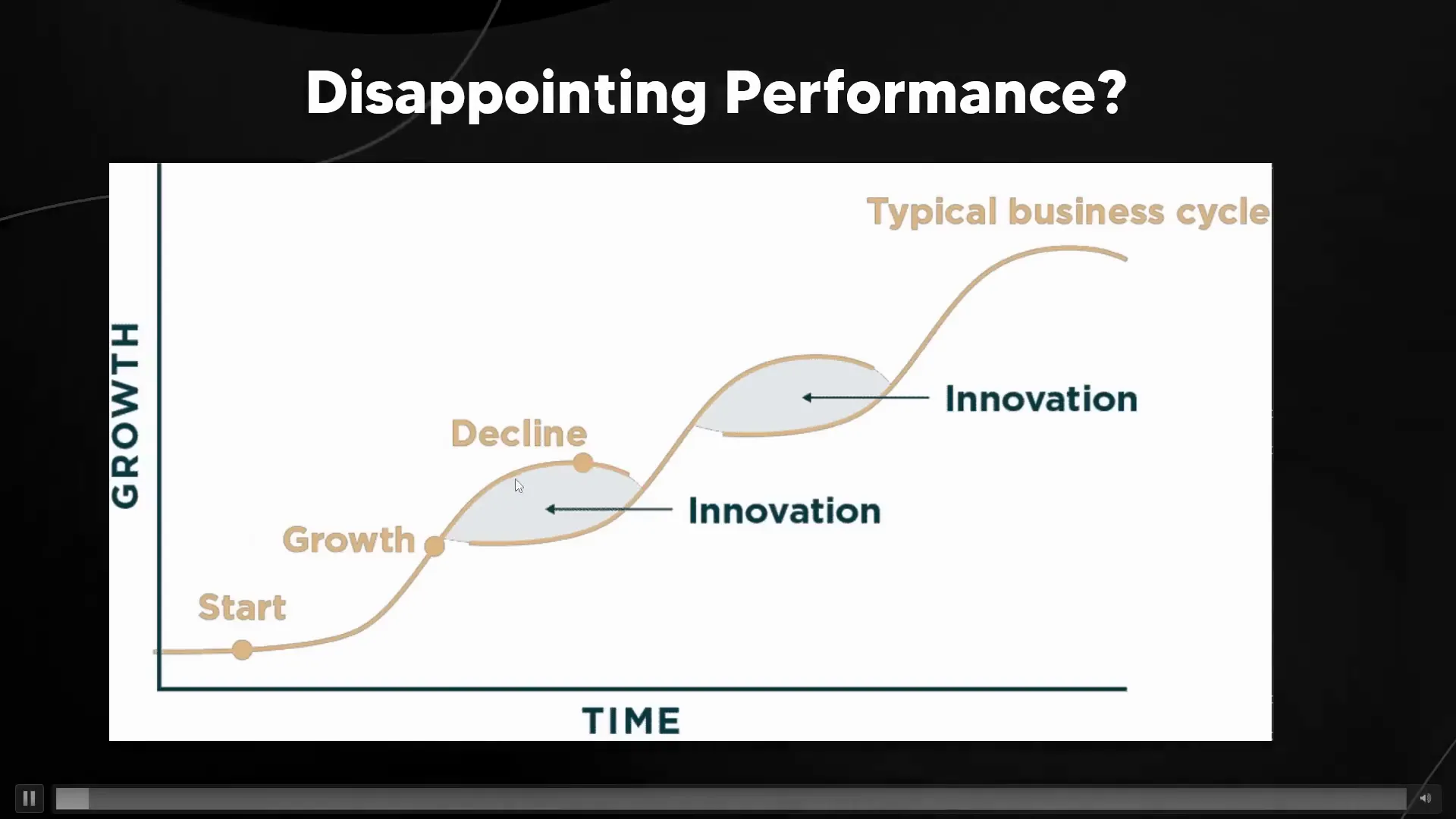
Understanding the S-Curve Dynamics
Initial Growth: Early AI models experienced rapid advancements, leading to a surge in capabilities.
Plateau Phase: We’re currently seeing a slowdown in significant improvements as scaling methods reach their limits.
Next Wave: Expect a resurgence as new paradigms and innovative approaches come into play, propelling AI into uncharted territories.
🚀 The Future of AI Paradigms
What does the future hold for AI? The landscape is ripe for transformation. As we shift our focus from scaling to discovery, we’re entering a new paradigm that emphasizes intelligence over size. This evolution could redefine our expectations of AI and its capabilities.
Imagine AI systems that not only process information but also understand context, nuance, and human emotions. This is the future we’re striving towards—a future where AI enhances human potential rather than merely mimicking it.
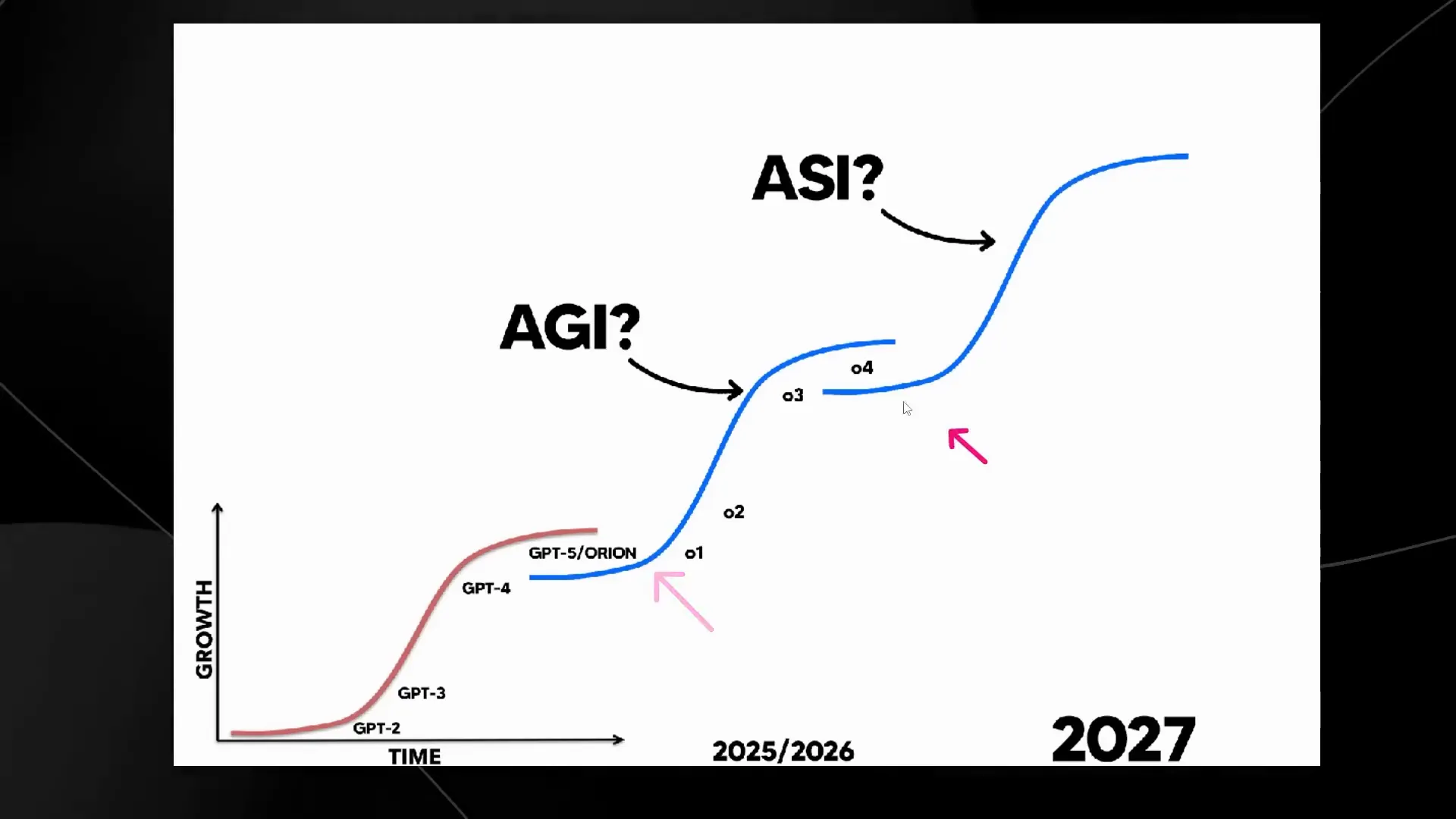
Anticipating Changes
Interdisciplinary Collaboration: The future will require collaboration across fields to foster innovative solutions.
Ethical Considerations: As AI becomes more capable, ethical considerations will play a crucial role in its development.
A Focus on Human-AI Interaction: Enhancing how AI systems interact with humans will be paramount to their success.
🧬 Defining Superintelligence
Superintelligence isn’t just a buzzword; it's a concept that could fundamentally alter our relationship with technology. It signifies an intelligence that surpasses the brightest human minds, capable of solving complex problems that currently baffle us.
This isn't just about creating smarter machines; it's about rethinking what intelligence means in the context of AI. Superintelligence could lead to breakthroughs in medicine, climate change, and even space exploration. The possibilities are limitless!
The Implications of Superintelligence
Solving Global Challenges: Superintelligence could tackle some of humanity's most pressing issues, from disease eradication to sustainable energy solutions.
Radical Improvements: If navigated correctly, superintelligence could enhance our quality of life in ways we can barely imagine.
Ethical Responsibility: With great power comes great responsibility. We must ensure that superintelligent systems align with human values and ethics.